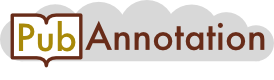
PMC:1481596 / 2943-4600
Annnotations
2_test
{"project":"2_test","denotations":[{"id":"16722537-10521342-1694478","span":{"begin":372,"end":373},"obj":"10521342"},{"id":"16722537-15284103-1694479","span":{"begin":474,"end":475},"obj":"15284103"},{"id":"16722537-15548452-1694480","span":{"begin":476,"end":477},"obj":"15548452"},{"id":"16722537-10591225-1694481","span":{"begin":506,"end":507},"obj":"10591225"},{"id":"16722537-12525261-1694482","span":{"begin":1099,"end":1101},"obj":"12525261"},{"id":"16722537-12538875-1694482","span":{"begin":1099,"end":1101},"obj":"12538875"},{"id":"16722537-12711690-1694482","span":{"begin":1099,"end":1101},"obj":"12711690"},{"id":"16722537-14517352-1694482","span":{"begin":1099,"end":1101},"obj":"14517352"},{"id":"16722537-12413400-1694482","span":{"begin":1099,"end":1101},"obj":"12413400"},{"id":"16722537-14576317-1694482","span":{"begin":1099,"end":1101},"obj":"14576317"},{"id":"16722537-12525261-1694483","span":{"begin":1121,"end":1123},"obj":"12525261"},{"id":"16722537-12538875-1694483","span":{"begin":1121,"end":1123},"obj":"12538875"},{"id":"16722537-12711690-1694483","span":{"begin":1121,"end":1123},"obj":"12711690"},{"id":"16722537-14517352-1694483","span":{"begin":1121,"end":1123},"obj":"14517352"},{"id":"16722537-14517352-1694484","span":{"begin":1272,"end":1274},"obj":"14517352"},{"id":"16722537-12413400-1694485","span":{"begin":1450,"end":1452},"obj":"12413400"},{"id":"16722537-14576317-1694486","span":{"begin":1453,"end":1455},"obj":"14576317"}],"text":"Protein interactions are routinely represented as graphs, with proteins as nodes and interactions as edges (links). Therefore, it is not surprising that analysis of protein interaction networks reach out for a variety of graph-theoretical tools. Following the observation that protein interaction networks display a characteristic power-law like node degree distribution [6], a substantial body of research focused on statistical properties of protein interaction networks [7,8]. In 1999, Hartwell et al. [9] introduced a notion of a functional module, a group of cellular components and their interaction that can be attributed a specific biological function. The authors also suggested the modular organization of molecular interaction networks, where each functional module involves a small number of cellular components and is autonomous, i.e., its interaction with other modules is limited to a few cellular components. Subsequently, this assumption was used in several computational methods to identify protein complexes and functional modules in high-throughput protein interaction networks [10-15]. Some methods [10-13] look for densely connected subgraphs within a protein interaction network, either cliques or \"cliquish\" components. For example, Spirin et al. [13] use the term functional module to denote groups of proteins which are densely connected within themselves but sparsely connected with the rest of the network. Other methods [14,15] combine protein interaction with other information to identify functional modules, such as signal transduction pathways, that do not necessarily correspond to densely connected regions of the network."}