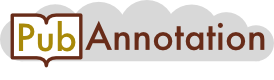
PMC:1459173 / 4502-13231
Annnotations
{"target":"https://pubannotation.org/docs/sourcedb/PMC/sourceid/1459173","sourcedb":"PMC","sourceid":"1459173","source_url":"https://www.ncbi.nlm.nih.gov/pmc/1459173","text":"Results and discussion\nSeveral measures of distance have been proposed and used to classify documents of diverse nature and to infer relationships among them. In practice, each measure translates in a computational task which might be more or less of a burden. In domains such as genome analysis and natural language processing, the increasing availability of longer and longer sequences and more and more massive data sets is playing havoc with similarity measures based on edit computations and the likes [2]. As an alternative, succinct scores related to compressibility -interpreted as a measure of structural complexity or information contents- have been deployed, of which the lineage may be traced back to Kolmogorov's complexity. The Kolmogorov complexity of a string x, denoted K(x), is the length of the shortest program that would cause a standard universal computer to output x. Along the same lines, the conditional Kolmogorov complexity K(x|y) for strings x and y is defined as the length of the shortest program that, given y as input, will output x as the result. Intuitively, the conditional complexity expresses the information difference between the strings x and y. We refer the reader to, e.g., [3] for a detailed treatment of the theory. Whereas the original Kolmogorov complexity is hardly computable, important emulators have been developed since [4], which conjugate compressibility and ease of computation. Following in these steps, we now test the discriminating power of the data compression method that is based on our Off-line steepest descent paradigm with extensible motifs.\nIn this paper, we present lossy off-line data compression techniques by textual substitution in which the patterns used in compression are chosen among the extensible motifs that are found to recur in the textstring with a minimum pre-specified frequency. Motif discovery and motif-driven parses of various kinds have been previously introduced and used in [5]. Whereas the motifs considered in those studies are \"rigid\", here we assume that each sequence of gaps present in a motif comes endowed with some individually prescribed degree of elasticity, whereby a same pattern may be stretched to fit segments of the source that match all the solid characters but are otherwise of different lengths. This is expected to save on the size of the codebook, and hence to improve compression.\nThe figure of compression achieved by our algorithm shows good sensitivity in telling apart veritable families of proteins from spurious blends. This sets forth an approach to classification that does away with alignment. The data used for the test consists of protein sequences, which are known to be hardly compressible at all [6]. The experiment reported below uses three different families which were picked at random from the PROSITE repository: AP endonucleases (acnucl), G-protein coupled receptors (gprot) and Succinyl-CoA ligases (succ). Table 2 summarizes the results of lossy and lossless compression for various values of the parameters. The artificial groups are marked \"-mix\", the last column shows the lossless compression ratio of fake over faithful families, when using motifs with the same parameter values. In all cases, the artificial families show compression ratios that are poorer by 10/20%, and the superiority of the lossy variants manifests itself throughout. The experiments thus verify the discrimination potential of data compression by extensible motifs. It seems thus meaningful to build a classifier on top of this measure. Compressibility by extensible motifs may be used to set up a similarity measure on sequences to be used in the inference of phylogeny. The measure could be extended into a metric distance, along the lines of [7]. Specifically, we denote by Off-line(z) the output size obtained when compressing a string z using the lossless variant of our paradigm, and compute the quantity:\nTable 1 The pseudocode of the motif extraction algorithm.\nMain() Iterate(m, B, Result)\n{ {\nResult ← {}; G:l m' ← m;\nB ← {mi|mi is a cell}; G:2 For each b = Extract(B) with\nFor each m = Extract(B) G:3 ((b ~-compatible m'\nIterate(m, B, Result); OR (m' ~-compatible b))\nResult ← Result; G:4 If (m' ~-compatible b)\n} G:5 mt ← m' ~ b;\nG:6 If Nodelnconsistent(mt) exit;\nG:7 If (|m'| = |b|) B ← B - {b};\nG:8 If (|| ≤ K)\nG:9 m' ← mt;\nG:10 Iterate(m', B, Result);\nG:11 If (b ~-compatible m')\nG:12 mt ← b ~ m';\nG:13 If Nodelnconsistent(mt) exit;\nG:14 If (|m'| = |b|) B ← B - {b};\nG:15 If (|| ≥ K)\nG:16 m' ← mt;\nG:17 Iterate(m', B, Result);\nG:18 For each r ∈ Result with r = m'\nG:19 If (m' is not maximal w.r.t. r) return;\nG:20 Result ← Result ⋃ {m'};\n}\nTable 2 Comparing sensitivity of lossy versus lossless compression by Off-line with Extensible Motifs, as applied to real and fake protein families.\nFile File len param density Lossy Lossless Compr ratio %\nK D\nacnucl 4197 10 3 1717 2425\n4197 5 3 1757 2370\nacnucl-mix 4149 10 3 1864 2629 +8.4\n4149 5 3 1972 2621 +10.6\ngprot 25482 30 3 7003 9879\n25482 20 3 7399 10276\ngprot-mix 25335 30 3 8179 11945 +20.9\n25335 20 3 8386 11978 +16.5\nsucc 16297 20 3 4994 7449\n16297 10 3 4977 7466\nsucc-mix 16410 20 3 5929 8803 +18.2\n16410 10 3 6415 8962 +19.2 where (xy) denotes the concatenation of x and y. Hence, D(x, y) measures the improvement over Off-line(y) that is brought about by using x as a \"dictionary\" when compressing y.\nIn the following experiment we construct a phylogeny of the Eutherian orders using complete unaligned mitochondrial genomes of the following 15 mammals from GenBank: human (Homo sapiens [GenBank:V00662]), chimpanzee (Pan troglodytes [GenBank:D38116]), pigmy chimpanzee (Pan paniscus [GenBank:D38113]), gorilla (Gorilla gorilla [GenBank:D38114]), orangutan (Pongo pygmaeus [GenBank:D38115]), gibbon (Hylobates lar [GenBank:X99256]), sumatran orangutan (Pongo pygmaeus abelii [GenBank:X97707]), horse (Equus caballus [GenBank:X79547]), white rhino (Ceratotherium simum [GenBank:Y07726]), harbor seal (Phoca vitulina [GenBank:X63726]), gray seal (Halichoerus grypus [GenBank:X72004]), cat (Felis catus [GenBank:U20753]), finback whale (Balenoptera physalus [GenBank:X61145]), blue whale (Balenoptera musculus [GenBank:X72204]), rat (Rattus norvegicus [GenBank:X14848]) and house mouse (Mus musculus [GenBank:V00711]).\nThe evolutionary tree in Figure 1 is generated by a variant of the classical neighbor-join where instead of minimizing the distances between nodes we maximized the separation. Specifically, for each pair (x, y) of sequences, the quantity D(x, y) is computed. Next, the neighbor-join algorithm is used to build the tree from the matrix of distances. This algorithms selects a pair of (x, y) among those achieving the minimum value for D, and creates an internal node as their father. It then coalesces x and y into a combined sequence the D value of which is computed as the maximum (instead of the average) of those of x and y. The process is continued until the D-matrix has shrunk to a scalar. The first notable finding is that closely related species are indeed grouped together, e.g., grayseal with harboseal, orangutan with sumatranorang, etc. Whereas there is no gold standard for the entire tree, biologists do suggest the following grouping for this case:\nFigure 1 The evolutionary tree built from complete mammalian mtDNA sequences of 15 species. [width = 450 pt]tree1.eps • Eutheria-Rodens: housemouse, rat.\n• Primates: chimpa, gibbon, gorilla, human, orangutan, pigmychimpa, sumatranorang.\n• Ferungulates: bluewhale, finbackwhale, grayseal, harboseal, horse, whiterhino.\nThe phylogeny obtained in our experiment is very close to the commonly accepted ones, which suggests that even a method of compression based on a single type of regularity, as opposed to those that take into account palindromes and other structures may support good comparative genomics.\nFor a comparison, the same treatment was applied to human language text classification, in analogy with what is found in [7,8]. Figure 2 displays the tree obtained in experiments performed with a small subset of languages on the widely translated \"Universal Declaration of Human Rights\". Once more, the resulting tree is coherent with commonly accepted ones.\nFigure 2 A partial tree of languages using a distance based on compression by extensible motifs. [width = 280 pt]tree2.eps","divisions":[{"label":"title","span":{"begin":0,"end":22}},{"label":"p","span":{"begin":23,"end":1606}},{"label":"p","span":{"begin":1607,"end":2393}},{"label":"p","span":{"begin":2394,"end":3924}},{"label":"table-wrap","span":{"begin":3925,"end":4973}},{"label":"label","span":{"begin":3925,"end":3932}},{"label":"caption","span":{"begin":3934,"end":3983}},{"label":"p","span":{"begin":3934,"end":3983}},{"label":"table","span":{"begin":3984,"end":4973}},{"label":"tr","span":{"begin":3984,"end":4013}},{"label":"td","span":{"begin":3984,"end":3990}},{"label":"td","span":{"begin":3992,"end":4013}},{"label":"tr","span":{"begin":4014,"end":4018}},{"label":"td","span":{"begin":4014,"end":4015}},{"label":"td","span":{"begin":4017,"end":4018}},{"label":"tr","span":{"begin":4019,"end":4048}},{"label":"td","span":{"begin":4019,"end":4031}},{"label":"td","span":{"begin":4033,"end":4048}},{"label":"tr","span":{"begin":4049,"end":4109}},{"label":"td","span":{"begin":4049,"end":4071}},{"label":"td","span":{"begin":4073,"end":4109}},{"label":"tr","span":{"begin":4110,"end":4165}},{"label":"td","span":{"begin":4110,"end":4133}},{"label":"td","span":{"begin":4135,"end":4165}},{"label":"tr","span":{"begin":4166,"end":4223}},{"label":"td","span":{"begin":4166,"end":4188}},{"label":"td","span":{"begin":4190,"end":4223}},{"label":"tr","span":{"begin":4224,"end":4281}},{"label":"td","span":{"begin":4224,"end":4240}},{"label":"td","span":{"begin":4242,"end":4281}},{"label":"tr","span":{"begin":4282,"end":4317}},{"label":"td","span":{"begin":4282,"end":4283}},{"label":"td","span":{"begin":4285,"end":4317}},{"label":"tr","span":{"begin":4318,"end":4367}},{"label":"td","span":{"begin":4318,"end":4367}},{"label":"tr","span":{"begin":4368,"end":4416}},{"label":"td","span":{"begin":4368,"end":4416}},{"label":"tr","span":{"begin":4417,"end":4448}},{"label":"td","span":{"begin":4417,"end":4448}},{"label":"tr","span":{"begin":4449,"end":4480}},{"label":"td","span":{"begin":4449,"end":4480}},{"label":"tr","span":{"begin":4481,"end":4528}},{"label":"td","span":{"begin":4481,"end":4528}},{"label":"tr","span":{"begin":4529,"end":4569}},{"label":"td","span":{"begin":4529,"end":4569}},{"label":"tr","span":{"begin":4570,"end":4603}},{"label":"td","span":{"begin":4570,"end":4603}},{"label":"tr","span":{"begin":4604,"end":4654}},{"label":"td","span":{"begin":4604,"end":4654}},{"label":"tr","span":{"begin":4655,"end":4704}},{"label":"td","span":{"begin":4655,"end":4704}},{"label":"tr","span":{"begin":4705,"end":4737}},{"label":"td","span":{"begin":4705,"end":4737}},{"label":"tr","span":{"begin":4738,"end":4770}},{"label":"td","span":{"begin":4738,"end":4770}},{"label":"tr","span":{"begin":4771,"end":4818}},{"label":"td","span":{"begin":4771,"end":4818}},{"label":"tr","span":{"begin":4819,"end":4868}},{"label":"td","span":{"begin":4819,"end":4868}},{"label":"tr","span":{"begin":4869,"end":4929}},{"label":"td","span":{"begin":4869,"end":4929}},{"label":"tr","span":{"begin":4930,"end":4971}},{"label":"td","span":{"begin":4930,"end":4971}},{"label":"tr","span":{"begin":4972,"end":4973}},{"label":"td","span":{"begin":4972,"end":4973}},{"label":"table-wrap","span":{"begin":4974,"end":5582}},{"label":"label","span":{"begin":4974,"end":4981}},{"label":"caption","span":{"begin":4983,"end":5123}},{"label":"p","span":{"begin":4983,"end":5123}},{"label":"table","span":{"begin":5124,"end":5582}},{"label":"tr","span":{"begin":5124,"end":5185}},{"label":"td","span":{"begin":5124,"end":5128}},{"label":"td","span":{"begin":5130,"end":5138}},{"label":"td","span":{"begin":5140,"end":5153}},{"label":"td","span":{"begin":5155,"end":5160}},{"label":"td","span":{"begin":5162,"end":5170}},{"label":"td","span":{"begin":5172,"end":5185}},{"label":"tr","span":{"begin":5186,"end":5190}},{"label":"td","span":{"begin":5186,"end":5187}},{"label":"td","span":{"begin":5189,"end":5190}},{"label":"tr","span":{"begin":5191,"end":5222}},{"label":"td","span":{"begin":5191,"end":5197}},{"label":"td","span":{"begin":5199,"end":5203}},{"label":"td","span":{"begin":5205,"end":5207}},{"label":"td","span":{"begin":5209,"end":5210}},{"label":"td","span":{"begin":5212,"end":5216}},{"label":"td","span":{"begin":5218,"end":5222}},{"label":"tr","span":{"begin":5223,"end":5245}},{"label":"td","span":{"begin":5223,"end":5227}},{"label":"td","span":{"begin":5229,"end":5230}},{"label":"td","span":{"begin":5232,"end":5233}},{"label":"td","span":{"begin":5235,"end":5239}},{"label":"td","span":{"begin":5241,"end":5245}},{"label":"tr","span":{"begin":5246,"end":5287}},{"label":"td","span":{"begin":5246,"end":5256}},{"label":"td","span":{"begin":5258,"end":5262}},{"label":"td","span":{"begin":5264,"end":5266}},{"label":"td","span":{"begin":5268,"end":5269}},{"label":"td","span":{"begin":5271,"end":5275}},{"label":"td","span":{"begin":5277,"end":5281}},{"label":"td","span":{"begin":5283,"end":5287}},{"label":"tr","span":{"begin":5288,"end":5317}},{"label":"td","span":{"begin":5288,"end":5292}},{"label":"td","span":{"begin":5294,"end":5295}},{"label":"td","span":{"begin":5297,"end":5298}},{"label":"td","span":{"begin":5300,"end":5304}},{"label":"td","span":{"begin":5306,"end":5310}},{"label":"td","span":{"begin":5312,"end":5317}},{"label":"tr","span":{"begin":5318,"end":5349}},{"label":"td","span":{"begin":5318,"end":5323}},{"label":"td","span":{"begin":5325,"end":5330}},{"label":"td","span":{"begin":5332,"end":5334}},{"label":"td","span":{"begin":5336,"end":5337}},{"label":"td","span":{"begin":5339,"end":5343}},{"label":"td","span":{"begin":5345,"end":5349}},{"label":"tr","span":{"begin":5350,"end":5375}},{"label":"td","span":{"begin":5350,"end":5355}},{"label":"td","span":{"begin":5357,"end":5359}},{"label":"td","span":{"begin":5361,"end":5362}},{"label":"td","span":{"begin":5364,"end":5368}},{"label":"td","span":{"begin":5370,"end":5375}},{"label":"tr","span":{"begin":5376,"end":5419}},{"label":"td","span":{"begin":5376,"end":5385}},{"label":"td","span":{"begin":5387,"end":5392}},{"label":"td","span":{"begin":5394,"end":5396}},{"label":"td","span":{"begin":5398,"end":5399}},{"label":"td","span":{"begin":5401,"end":5405}},{"label":"td","span":{"begin":5407,"end":5412}},{"label":"td","span":{"begin":5414,"end":5419}},{"label":"tr","span":{"begin":5420,"end":5452}},{"label":"td","span":{"begin":5420,"end":5425}},{"label":"td","span":{"begin":5427,"end":5429}},{"label":"td","span":{"begin":5431,"end":5432}},{"label":"td","span":{"begin":5434,"end":5438}},{"label":"td","span":{"begin":5440,"end":5445}},{"label":"td","span":{"begin":5447,"end":5452}},{"label":"tr","span":{"begin":5453,"end":5483}},{"label":"td","span":{"begin":5453,"end":5457}},{"label":"td","span":{"begin":5459,"end":5464}},{"label":"td","span":{"begin":5466,"end":5468}},{"label":"td","span":{"begin":5470,"end":5471}},{"label":"td","span":{"begin":5473,"end":5477}},{"label":"td","span":{"begin":5479,"end":5483}},{"label":"tr","span":{"begin":5484,"end":5508}},{"label":"td","span":{"begin":5484,"end":5489}},{"label":"td","span":{"begin":5491,"end":5493}},{"label":"td","span":{"begin":5495,"end":5496}},{"label":"td","span":{"begin":5498,"end":5502}},{"label":"td","span":{"begin":5504,"end":5508}},{"label":"tr","span":{"begin":5509,"end":5550}},{"label":"td","span":{"begin":5509,"end":5517}},{"label":"td","span":{"begin":5519,"end":5524}},{"label":"td","span":{"begin":5526,"end":5528}},{"label":"td","span":{"begin":5530,"end":5531}},{"label":"td","span":{"begin":5533,"end":5537}},{"label":"td","span":{"begin":5539,"end":5543}},{"label":"td","span":{"begin":5545,"end":5550}},{"label":"tr","span":{"begin":5551,"end":5582}},{"label":"td","span":{"begin":5551,"end":5556}},{"label":"td","span":{"begin":5558,"end":5560}},{"label":"td","span":{"begin":5562,"end":5563}},{"label":"td","span":{"begin":5565,"end":5569}},{"label":"td","span":{"begin":5571,"end":5575}},{"label":"td","span":{"begin":5577,"end":5582}},{"label":"p","span":{"begin":5584,"end":5760}},{"label":"p","span":{"begin":5761,"end":6675}},{"label":"p","span":{"begin":6676,"end":7639}},{"label":"figure","span":{"begin":7640,"end":7758}},{"label":"label","span":{"begin":7640,"end":7648}},{"label":"caption","span":{"begin":7650,"end":7758}},{"label":"p","span":{"begin":7650,"end":7758}},{"label":"p","span":{"begin":7759,"end":7794}},{"label":"p","span":{"begin":7795,"end":7877}},{"label":"p","span":{"begin":7878,"end":7958}},{"label":"p","span":{"begin":7959,"end":8246}},{"label":"p","span":{"begin":8247,"end":8605}},{"label":"label","span":{"begin":8606,"end":8614}}],"tracks":[{"project":"2_test","denotations":[{"id":"16722593-12611807-1693591","span":{"begin":508,"end":509},"obj":"12611807"},{"id":"16722593-11238070-1693592","span":{"begin":8371,"end":8372},"obj":"11238070"}],"attributes":[{"subj":"16722593-12611807-1693591","pred":"source","obj":"2_test"},{"subj":"16722593-11238070-1693592","pred":"source","obj":"2_test"}]}],"config":{"attribute types":[{"pred":"source","value type":"selection","values":[{"id":"2_test","color":"#ecb193","default":true}]}]}}