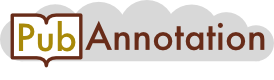
PMC:1247194 / 42028-51904
Annnotations
{"target":"https://pubannotation.org/docs/sourcedb/PMC/sourceid/1247194","sourcedb":"PMC","sourceid":"1247194","source_url":"https://www.ncbi.nlm.nih.gov/pmc/1247194","text":"Discussion\nOur findings indicate that GIS can greatly enhance epidemiologic research in terms of definition of source and routes of potential exposure and estimation of environmental levels of target contaminants in the exposure assessment process. We found over 15 studies published since 1998 that describe the successful use of GIS for one or more of these purposes. Across all of these studies, there was consensus that the use of GIS was instrumental in achieving optimal exposure assessment. In our example studies, GIS improved resolution of the source of potential exposure (Elliott et al. 2001; Reif et al. 2003), identified the most likely route of exposure (Reif et al. 2003), and estimated levels of target contaminants for use in estimating exposure to the study population (Nyberg et al. 2000; Reif et al. 2003). Our examples of environmental epidemiology studies using GIS also emphasize the importance of interdisciplinary study teams.\nGIS have been used to evaluate environmental justice issues, usually by linking information about potential sources of environmental pollutants to census information on sociodemographic characteristics of a population (Perlin et al. 2001; Waller et al. 1999). However, only recently have GIS been used in the design of environmental epidemiology studies. Each example in our article demonstrates that GIS can (and perhaps should) be used in the early planning stages of an environmental epidemiology study to help locate a potential study population with a wide range of exposure. The statistical power of an epidemiologic study and the precision of the risk estimates are optimized when the study population includes adequate numbers of those with both high and low exposures. An example of how GIS have been used to identify a study population with a range of exposures is a feasibility study of childhood leukemia and electromagnetic radiation from power transmission lines in New Jersey (Wartenberg et al. 1993). A GIS was used to identify the population living close to transmission lines and a comparison population farther away. Demographic information was evaluated for both the exposed and unexposed populations to determine potential confounding factors. Other examples include the use of GIS for surveillance and study of lead poisoning from residential exposures (Roberts et al. 2003; Wartenberg 1992).\nThe increasing availability of environmental databases in a geographic format (Paulu et al. 1995), including the location of industrial sites and releases (Toxic Release Inventory Program 2004), should make it feasible to incorporate these potential exposure data into epidemiologic studies. For example, in a recently started cross-sectional study on potential adverse health effects (primarily hypertension) of airport-related noise exposure, study populations are being selected using modeled noise contours around the participating airports (European Commission 2003). Such models are particularly applicabile in the selection of study populations exposed to different levels of the pollutants under study, using a cross-sectional or cohort study approach. A case–control design, in which cases are selected from, for example, hospital data or cancer registries, will usually have a predefined area (hospital catchment or cancer registry area); thus, preexisting exposure information may be less relevant in the study population selection. However, exposure information can be used to delimit the study area within the bounds of the catchment area or disease registry. For example, AWWA (2000) demonstrated the feasibility of linking environmental monitoring data with birth and cancer registry data to identify optimal geographic locations for epidemiologic studies of by-products of chlorination in public water supplies in the United States. GIS also have potential uses in the selection of controls for an epidemiologic study, as they are usually randomly selected from the same geographic area as the cases. As frequency matching (on age and sex) is commonly applied for study efficiency reasons, GIS could also be used for further frequency matching on SES, where areas are classified according to a georeferenced SES index.\nThere are, of course, a number of caveats regarding use of GIS for exposure assessment in environmental epidemiologic studies. We reviewed fundamental principles of three scientific disciplines critical to such applications: geospatial science, environmental science, and epidemiology. Axiomatic themes from each of these scientific disciplines should be adhered to in any case, but they are particularly relevant when using a GIS. These themes include accuracy and validity of data (raw and calculated), appropriate selection of mathematic formulas and models, and scientific plausibility. The application of these axiomatic themes can be very different across the scientific disciplines, which reinforces the need for multidisciplinary teams in conducting environmental epidemiology studies. For example, researchers in each of the disciplines are trained in determining the accuracy and precision of measurement data. However, only the geospatial scientist or geographer is generally trained to rectify geographic data so that two or more GIS-based data layers such as health outcome and environmental data can be merged and the resulting data layer used to determine the association more accurately. Similarly, only the epidemiologist is likely to be trained to search for and identify other data layers that, if omitted from the test of association, could confound the results.\nUse of measured environmental data and mathematic algorithms for estimating contaminant levels in exposure assessment is another area requiring specialized expertise in most cases. Since the advent of the computer age, packaged software has become more and more prevalent for such applications, but the old modeler adage “garbage in, garbage out” is perpetual truth. Even with the color maps produced using a GIS, “mapped garbage” is still “garbage.” In this article we propose several fundamental principles of environmental science and modeling that should be adhered to when using GIS in exposure assessment for epidemiology studies. Perhaps the most important of these principals can be captured by the term “validation.” In each of our example studies, environmental data were used to develop an exposure metric for use in epidemiology. The data used were collected for other purposes, commonly for administrative or regulatory use. These studies demonstrate the range of measurement data quality and degree of validation that may be possible from relatively low (Elliott et al. 2001) to high (Nyberg et al. 2000). They also demonstrate the likely consequences across this range in terms of risk estimates in an epidemiology study. In Elliott et al. (2001), a database on landfill sites was obtained from the environmental protection agencies, which collected the data from site operators in the licensing process. Thus, data that would have been useful for exposure assessment were not readily available (e.g., volumes and types of waste actually received at the landfill sites, measurement data for specific chemicals being released into the environment, or the extent of contamination). Instead, the likely limit of dispersion for landfill emissions (2 km) was estimated based on published information and used as an exposure boundary around each site, degree of hazard for exposure was derived from the type of license held by the operator, and the epidemiologic analysis assumed a common relative risk for all landfill sites. The researchers did not validate these exposure metrics. It is likely that sites licensed to carry special (hazardous) waste did not necessarily do so, and that sites licensed to carry nonspecial waste actually did carry some hazardous waste as well. The resulting exposure misclassification was most likely nondifferential, which could result in a bias risk estimate toward the null (Copeland et al. 1977). The findings of the study, small excess risks for some birth outcomes after exposure to landfills, seem to verify this conclusion.\nThe study reported by Reif et al. (2003) concerning TCE and neurobehavioral demonstrated that improvement in exposure assessment techniques “refined exposure . . . with adequate specificity to reveal adverse effects [of TCE] in the nervous system.” In that study, the researchers refined exposure assessment by replacing a proximity metric such as the one used in Elliott et al. (2001) with exposure predictions based on validated environmental measurements (TCE levels in groundwater at source wells for a municipal water system) and validated transport modeling (water pressure and volume in the municipal water system) during the exposure period for the study. However, data were not available to validate predicted TCE levels at study participants’ residences.\nIn the final example study that we reviewed, Bellander et al. (2001) had sufficient source emission and environmental measurement data to calibrate and validate predicted levels of NO2 in the environment of Stockholm, Sweden, for at least a portion of the exposure period in an epidemiologic study of lung cancer (1955–1990). They also validated their predicted location of residence in Stockholm for each participant in the study by cross-checking results using external geocoding service companies. The resolution and precision of this exposure assessment process resulted in the capability to detect a wide range of individual long-term average exposure and to detect risk of lung cancer to average traffic level exposure to NO2 within a 95% confidence limit. The procedures and results of these studies clearly indicate the need for expertise in environmental science and related disciplines in epidemiologic studies involving pollutant emissions.","divisions":[{"label":"title","span":{"begin":0,"end":10}},{"label":"p","span":{"begin":11,"end":951}},{"label":"p","span":{"begin":952,"end":2366}},{"label":"p","span":{"begin":2367,"end":4201}},{"label":"p","span":{"begin":4202,"end":5584}},{"label":"p","span":{"begin":5585,"end":8159}},{"label":"p","span":{"begin":8160,"end":8924}}],"tracks":[{"project":"2_test","denotations":[{"id":"15198921-11509424-28170904","span":{"begin":598,"end":602},"obj":"11509424"},{"id":"15198921-14615234-28170905","span":{"begin":616,"end":620},"obj":"14615234"},{"id":"15198921-14615234-28170906","span":{"begin":681,"end":685},"obj":"14615234"},{"id":"15198921-14615234-28170907","span":{"begin":820,"end":824},"obj":"14615234"},{"id":"15198921-11266104-28170908","span":{"begin":1185,"end":1189},"obj":"11266104"},{"id":"15198921-10189627-28170909","span":{"begin":1205,"end":1209},"obj":"10189627"},{"id":"15198921-8143596-28170910","span":{"begin":1962,"end":1966},"obj":"8143596"},{"id":"15198921-12766217-28170911","span":{"begin":2343,"end":2347},"obj":"12766217"},{"id":"15198921-1464284-28170912","span":{"begin":2360,"end":2364},"obj":"1464284"},{"id":"15198921-8570834-28170913","span":{"begin":2459,"end":2463},"obj":"8570834"},{"id":"15198921-11509424-28170914","span":{"begin":6669,"end":6673},"obj":"11509424"},{"id":"15198921-11509424-28170915","span":{"begin":6841,"end":6845},"obj":"11509424"},{"id":"15198921-871121-28170916","span":{"begin":8022,"end":8026},"obj":"871121"},{"id":"15198921-14615234-28170917","span":{"begin":8195,"end":8199},"obj":"14615234"},{"id":"15198921-11509424-28170918","span":{"begin":8540,"end":8544},"obj":"11509424"},{"id":"15198921-11445519-28170919","span":{"begin":8988,"end":8992},"obj":"11445519"}],"attributes":[{"subj":"15198921-11509424-28170904","pred":"source","obj":"2_test"},{"subj":"15198921-14615234-28170905","pred":"source","obj":"2_test"},{"subj":"15198921-14615234-28170906","pred":"source","obj":"2_test"},{"subj":"15198921-14615234-28170907","pred":"source","obj":"2_test"},{"subj":"15198921-11266104-28170908","pred":"source","obj":"2_test"},{"subj":"15198921-10189627-28170909","pred":"source","obj":"2_test"},{"subj":"15198921-8143596-28170910","pred":"source","obj":"2_test"},{"subj":"15198921-12766217-28170911","pred":"source","obj":"2_test"},{"subj":"15198921-1464284-28170912","pred":"source","obj":"2_test"},{"subj":"15198921-8570834-28170913","pred":"source","obj":"2_test"},{"subj":"15198921-11509424-28170914","pred":"source","obj":"2_test"},{"subj":"15198921-11509424-28170915","pred":"source","obj":"2_test"},{"subj":"15198921-871121-28170916","pred":"source","obj":"2_test"},{"subj":"15198921-14615234-28170917","pred":"source","obj":"2_test"},{"subj":"15198921-11509424-28170918","pred":"source","obj":"2_test"},{"subj":"15198921-11445519-28170919","pred":"source","obj":"2_test"}]},{"project":"MyTest","denotations":[{"id":"15198921-11509424-28170904","span":{"begin":598,"end":602},"obj":"11509424"},{"id":"15198921-14615234-28170905","span":{"begin":616,"end":620},"obj":"14615234"},{"id":"15198921-14615234-28170906","span":{"begin":681,"end":685},"obj":"14615234"},{"id":"15198921-14615234-28170907","span":{"begin":820,"end":824},"obj":"14615234"},{"id":"15198921-11266104-28170908","span":{"begin":1185,"end":1189},"obj":"11266104"},{"id":"15198921-10189627-28170909","span":{"begin":1205,"end":1209},"obj":"10189627"},{"id":"15198921-8143596-28170910","span":{"begin":1962,"end":1966},"obj":"8143596"},{"id":"15198921-12766217-28170911","span":{"begin":2343,"end":2347},"obj":"12766217"},{"id":"15198921-1464284-28170912","span":{"begin":2360,"end":2364},"obj":"1464284"},{"id":"15198921-8570834-28170913","span":{"begin":2459,"end":2463},"obj":"8570834"},{"id":"15198921-11509424-28170914","span":{"begin":6669,"end":6673},"obj":"11509424"},{"id":"15198921-11509424-28170915","span":{"begin":6841,"end":6845},"obj":"11509424"},{"id":"15198921-871121-28170916","span":{"begin":8022,"end":8026},"obj":"871121"},{"id":"15198921-14615234-28170917","span":{"begin":8195,"end":8199},"obj":"14615234"},{"id":"15198921-11509424-28170918","span":{"begin":8540,"end":8544},"obj":"11509424"},{"id":"15198921-11445519-28170919","span":{"begin":8988,"end":8992},"obj":"11445519"}],"namespaces":[{"prefix":"_base","uri":"https://www.uniprot.org/uniprot/testbase"},{"prefix":"UniProtKB","uri":"https://www.uniprot.org/uniprot/"},{"prefix":"uniprot","uri":"https://www.uniprot.org/uniprotkb/"}],"attributes":[{"subj":"15198921-11509424-28170904","pred":"source","obj":"MyTest"},{"subj":"15198921-14615234-28170905","pred":"source","obj":"MyTest"},{"subj":"15198921-14615234-28170906","pred":"source","obj":"MyTest"},{"subj":"15198921-14615234-28170907","pred":"source","obj":"MyTest"},{"subj":"15198921-11266104-28170908","pred":"source","obj":"MyTest"},{"subj":"15198921-10189627-28170909","pred":"source","obj":"MyTest"},{"subj":"15198921-8143596-28170910","pred":"source","obj":"MyTest"},{"subj":"15198921-12766217-28170911","pred":"source","obj":"MyTest"},{"subj":"15198921-1464284-28170912","pred":"source","obj":"MyTest"},{"subj":"15198921-8570834-28170913","pred":"source","obj":"MyTest"},{"subj":"15198921-11509424-28170914","pred":"source","obj":"MyTest"},{"subj":"15198921-11509424-28170915","pred":"source","obj":"MyTest"},{"subj":"15198921-871121-28170916","pred":"source","obj":"MyTest"},{"subj":"15198921-14615234-28170917","pred":"source","obj":"MyTest"},{"subj":"15198921-11509424-28170918","pred":"source","obj":"MyTest"},{"subj":"15198921-11445519-28170919","pred":"source","obj":"MyTest"}]}],"config":{"attribute types":[{"pred":"source","value type":"selection","values":[{"id":"2_test","color":"#ecb493","default":true},{"id":"MyTest","color":"#9a93ec"}]}]}}