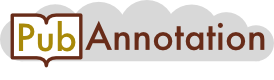
@ewha-bio:288
Annnotations
{"target":"https://pubannotation.org/docs/sourcedb/@ewha-bio/sourceid/288","sourcedb":"@ewha-bio","sourceid":"288","text":"\n=============Title==========\nCopy Number Variations in the Human Genome: Potential Source for Individual Diversity and Disease Association Studies.\n=============Cor Author==========\n*Corresponding author: E-mail yejun@catholic.ac.krTel +82-2-590-1214, Fax +82-2-596-8969 Accepted 11 March 2008\n===========Author==========\nTae-Min Kim1, Seon-Hee Yim2 and Yeun-Jun Chung1,2*1Department of Microbiology, 2Integrated Research Center for Genome Polymorphism, The Catholic University of Korea, Seoul 137-701, Korea\n===========Keywords==========\nKeywords: array-CGH, Copy number variation (CNV), Genome-wide association study (GWAS)Keywords: chromosome, genome-wide linkage search, heritability, HDL cholesterolKeywords: inbreeding coefficient, Mengolian population, STR, HWE, PICKeywords: haplotype, HapMap, Korean, LD, populations, SNP\n===========Sub Heading==========\nAbstract\tIntroduction\tThe definition of CNV\tThe identification of CNVs using differ-ent platforms\tClinical implications of CNVs and dis-ease association study\tConclusion\tIntroduction\tMethods\tResults and Discussion\tIntroduction\tMethods\tResults\tDiscussion\tIntroduction\tMethods\tMethods\tConstruction of Adenoviral Vector for hTERT-specific Group I Intron \tMajor Functionalities of CGHscape\tMethods\tFeatures and Results\tPersonal Genomics\tPolymorphism and Mutation Databases\tMethods\tFeatures and Results\tMethods \tFeatures and Results\tMethodology\t\n==========Minor Heading===========\nASubjects, medical histories, genotyping, and measurement of HDL cholesterol\tStatistical analyses, heritability estimation, and variance component linkage analysis\tParticipants\tGenotyping\tEstimating Hardy-Weinberg Equilibrium (HWE), Information Contents and Inbreeding Coefficients\tASNP Selection\tDNA Samples\tGenotyping\tStatistical Analysis \tADatasets\tThe dataset\tSubjects\tMethod\tDataset\t\n===========Main Text==========\n\n\nAbstract.\n\n\n\nThe widespread presence of large-scale genomic variations, termed copy number variation (CNVs), has been recently recognized in phenotypically normal individuals.\n\n\n\nJudging by the growing number of reports on CNVs, it is now evident that these variants contribute significantly to genetic diversity in the human genome.\n\n\n\nLike single nucleotide polymorphisms (SNPs), CNVs are expected to serve as potential biomarkers for disease susceptibility or drug responses.\n\n\n\nHowever, the technical and practical concerns still remain to be tackled.\n\n\n\nIn this review, we examine the current status of CNV DBs and research, including the ongoing efforts of CNV screening in the human genome.\n\n\n\nWe also discuss the characteristics of platforms that are available at the moment and suggest the potential of CNVs in clinical research and application.\n\n\n\nIIntroduction.\n\n\n\nTraditionally, large-scale genomic variants that are visible in conventional karyotyping have been thought to be associated with early-onset, highly penetrant genetic disorders, while they are incompatible in normal, disease-free individuals (Lupski, 1998; Stankiewicz and Lupski, 2002).\n\n\n\nThe construction of the 'reference genome' by the human genome sequencing project is based on the belief that human genome sequences are virtually identical, even in different individuals, except for well-known single nucleotide polymorphisms (SNP) or size-variants of tandem repeats such as mini- or microsatellites (variable number of tandem repeats or VNTR) (Przeworski et al., 2000).\n\n\n\nThis traditional concept has been recently challenged by the discovery that large structural variations are more prevalent than previously presumed (Check, 2005).\n\n\n\nUsing high-resolution whole- genome scanning technologies such as array-based comparative genomic hybridization (array-CGH), two groups of pioneering scientists have identified widespread copy number variations (CNVs) in apparently healthy, normal individuals (Iafrate et al., 2004; Sebat et al., 2004).\n\n\n\nIt proposes that our genome is more diverse than has ever been recognized, and subsequent studies have identified up to 11,000 CNVs across the whole genome (Tuzun et al., 2005; Hinds et al., 2006; Mills et al., 2006; McCarroll et al., 2006; Conrad et al., 2006; Sharp et al., 2005; Wong et al., 2007; de Smith et al., 2007).\n\n\n\nAlthough the current understanding of CNVs is still limited for practical use and technical challenges still remain to be tackled, recent studies already have demonstrated the potential association of CNVs with various diseases, suggesting plausible functional significances and highlighting the promising utility of CNVs.\n\n\n\nThe current coverage of CNVs in the human genome already has exceeded that of SNPs (approximately 600 Mb comprising 12% of human genome) and is still increasing (Cooper et al., 2007).\n\n\n\nThese large-scale structural variants, in addition to SNPs, will serve as powerful sources to help our understanding of human genetic variation and of differences in disease susceptibility for various diseases.\n\n\n\nThis paper reviews the current knowledge and future perspectives of CNVs.\n\n\n\nThe definition of CNV.\n\n\n\nStructural variations that involve large DNA segments can take various forms, such as duplication, deletion, insertion, inversion, and translocation.\n\n\n\nAmong them, DNA copy number variations larger than 1 kb are collectively termed CNVs.\n\n\n\nFig.\n\n\n\n1 illustrates the concept of CNV.\n\n\n\nAlthough the CNV can include large, microscopically visible genomic variations, it generally indicates a submicroscopic structural variation that is hardly detectable by conventional karyotyping (35 Mb) (Freeman et al., 2006).\n\n\n\nSmaller variations such as small insertional- deletion (indel) polymorphisms are not included in CNVs, while they comprise another large collection of over 400,000 variants in the human genome (Mills et al., 2006), and neither is the insertional polymorphism of mobile elements such as Alus or L1 elements considered a CNV.\n\n\n\nAt the beginning stages of CNV discovery, a number of terms were proposed to define them e.g., large-scale copy number variants (LCV) (Iafrate et al., 2004), copy number polymorphism (CNP) (Sebat et al., 2004), and intermediate-sized variants (ISV) (Tuzun et al., 2005).\n\n\n\nThe current definition of CNV is also operational and can be modified with the advance of scanning resolution and coverage, and availability of allele frequency in a determined population.The identification of CNVs using differ-ent platforms.\n\n\n\nVarious scanning platforms and quality control methods have been used to identify CNV calls.\n\n\n\nBecause the choice of platforms has a great effect on the results, it is worth reviewing the characteristics of platforms to improve the understanding of CNVs.\n\n\n\nThe presence of CNVs in normal individuals was reported for the first time in 2004 independently by two groups led by Lee C. and Wigler M. (Iafrate et al., 2004; Sebat et al., 2004).\n\n\n\nBoth studies used two-dye array-CGH techniques that used clones of bacterial artificial chromosomes (BAC) or oligonucleotides (representational oligonucleotide microarray analysis, or ROMA).\n\n\n\nTheyindependently reported about 250 and 80 loci as changes in copy number from 39 and 20 normal individuals, respectively.\n\n\n\nFig.\n\n\n\n2 illustrates the general concept of CNV detection based on two-dye array-CGH.\n\n\n\nAlthough the average numbers of CNVs per individual genome were similar in two studies (about 12 CNVs per genome), it should be noted that there was little overlap between the results.\n\n\n\nThis discrepancy between studies was possibly due to the use of different platforms and experimental conditions in different populations.\n\n\n\nHowever, it is also probable that there are still large numbers of structural variants that have yet to be discovered (Buckley et al., 2005; Eichler, 2006).\n\n\n\nOne following study that provided evidence on the widespread presence of large-scale structural variations in the human genome was based solely on in silico analysis (Tuzun et al., 2005).\n\n\n\nThe sequence-level comparison of two independent genome sequences, i.e., one derived from a human genome reference assembly and the other from fosmid clones of a genomic library, revealed about 300 structural variations, including inversions.\n\n\n\nThis method can detect various types of structural variants, including inversion, which is not detectable by conventional array-CGH platforms.\n\n\n\nIndeed, the results by Tuzun et al.\n\n\n\n(2005) can be used as validated control for primary verification or for parameter tuning for the development of CNV-detection platforms or algorithms.\n\n\n\nAlthough the use of this method is currently limited by the unavailability of sequence data, ongoing efforts to sequence the individual human genome and to develop cost-effective sequencing platforms (Bennett et al., 2005) will be able to facilitate sequence-level genome comparisons and the identification of highly qualified structural variants in the near future.\n\n\n\nTwo studies by McCarroll et al.\n\n\n\nand Conrad et al., which focused on the identification of deletion variants (McCarroll et al., 2006; Conrad et al., 2006), used 1.2 million SNP genotyping data from The International HapMap Consortium (International HapMap Consortium.\n\n\n\n2005).\n\n\n\nThey assumed that allelic deletion causes the discard of probes in SNP genotyping.\n\n\n\nFor example, the runs of consecutive probes with null genotype calls or runs of SNP genotypes whose allelic frequencies deviate from expected Hardy-Weinberg equilibrium ratios or expected Mendelian inheritance patterns might represent the presence of deleted loci.\n\n\n\nThey independently reported about 600 potential deletions as small as less than 100 bp.\n\n\n\nThe relatively small size of the identified variants, compared with the array-CGH method, is due to the high resolution of the platforms.\n\n\n\nThe use of an SNP-centric array platform can be used to identify linkage disequilibrium (LD) of structural variants with nearby SNPs in a given population.\n\n\n\nBut, the discrepancy in deletions that were identified in the two studies was also noted in spite of using similar HapMap populations and identification methods (Eichler 2006).\n\n\n\nRecently, a comprehensive CNV analysis was reported based on high-resolution array platforms, Whole Genome TilePath (WGTP), which used 26,000 large insert clones, and Affymetric GeneChip Human Mapping 500K early access, which used 500,000 SNP oligonucleotides.\n\n\n\nThey identified about 1500 genomic segments as copy number variations or CNVRs (copy number variable regions) consisting of overlapping CNVs from 269 HapMap individuals (Redon et al., 2006).\n\n\n\nThe results from the two platforms are worth comparing becasuse they provide the highest currently achievable resolution and are often selected as primary platforms in many other studies.\n\n\n\nFirstly, the CNVs that are identified from BAC-based array-CGH are generally larger than those from oligonucleotide-based arrays (230 kb and 80 kb of median size, respectively).\n\n\n\nThis overestimation of CNVs by BAC-based array-CGH is due to the large insert clones that are used, which has been frequently reported (Iafrate et al., 2006).\n\n\n\nSecondly, the actual boundaries of structural variants can not be determined through BAC-based array-CGH.\n\n\n\nOn the other hand, a more accurate determination of variant boundaries can be achieved through SNP-centric oligonucleotide-based arrays that have an extensive number of oligonucleotides.\n\n\n\nThe SNP-centric platform has additional advantages of accompanying SNP genotype information as a potential variant source, combined with large structural variants and its ability to detect the presence of loss of heterozygosity (LOH) or segmental uniparental disomy (Bruce et al., 2005; Mei et al., 2000).\n\n\n\nBut, the SNP-centric platform also has its disadvantages.\n\n\n\nIn spite of the advanced resolution, the relatively low signal-to-noise ratio of oligonucleotide-based hybridization intensity, compared with large insert clone array, might result in higher false-positive rates.\n\n\n\nBecause most CNVs are subtle changes, this makes the results prone to misclassification of signal intensities and, consequently, to statistical errors.\n\n\n\nSometimes, it is pointed out that the SNP-centric array was originally designed for allelic discrimination and is not appropriate for CNV detection because of biased genomic distribution and sequence composition of spotted probes (McCarroll and Altshuler 2007d).\n\n\n\nRecently proposed oligonucleotide-based array platforms have been designed for CNV detection specifically without sacrificing the advantage of high resolution, which can be a promising solution for CNV detection in the near future (Barrett et al., 2004).\n\n\n\nIn identifying CNVs in normal populations, one of the fundamental problems is the lack of a reference genome from which diploid states of sample DNA can be inferred.\n\n\n\nUnlike the array-CGH-based tumor study in which the normal DNA of the same individual can be used as a reference genome, no single DNA source can present the standardized and universal genome in variant analysis.\n\n\n\nOften, the pooled genome of several individuals has been used to represent the average genome, while the heterogeneity of the used population might affect the copy number inference step, as shown for examples of X chromosomes.\n\n\n\nRedon et al.\n\n\n\nand Komura et al.\n\n\n\nadopted the pairwise comparison for ac-curate inference of copy number states in individual loci, which is noteworthy (Redon et al., 2006; Komura et al., 2006).\n\n\n\nIn pairwise comparison, the hybridization intensities of one sample is compared with those of all other remaining samples as one large reference, and the diploid states of loci can be more accurately inferred from the multiple comparison results.Clinical implications of CNVs and dis-ease association study.\n\n\n\nIn spite of recent technological developments of genetic polymorphism-oriented disease association studies, still little is known about the effects of genetic polymorphisms on common complex diseases.\n\n\n\nOne of the ultimate goals in exploring CNVs is to systematically assess the association between such variants and the disease.\n\n\n\nAlthough it is unlikely that all CNVs in the human genome are associated with diseases, evidence of the association of CNVs and a wide spectrum of human diseases has rapidly accumulated.\n\n\n\nTable 1 summarizes the CNVs that have been reported to be associated with diseases.\n\n\n\nCNVs can affect disease susceptibility or individual differences in responses to drugs through alteration of gene expression.\n\n\n\nStranger et al.\n\n\n\n's and Heidenblad et al's reports coherently showed positive correlations between DNA copy number dosage and gene expression level (Stranger et al., 2007; Heidenblad et al., 2005).\n\n\n\nIf a CNV region contains transcriptional regulatory elements rather than protein coding genes, it still can affect gene expression levels by changing transcriptional regulation or heterochromatin spread (Reymond et al., 2007).Conclusion.\n\n\n\nThe genomic fraction that is occupied by CNVs is now estimated to be about 600 Mb, already exceeding that of single base-level variants.\n\n\n\nIt is likely that the number of CNVs and the genomic fraction that is affected by structural variants will continue to expand, and many of them will be used for more practical purposes, including disease association or population studies.\n\n\n\nHowever, it should be remembered that the current CNV entries are plagued by substantial amounts of false-positive and false-negative results.\n\n\n\nOnly a small portion of them have been validated by independent methods.\n\n\n\nTo overcome this, it is necessary to improve scanning platforms, including optimizing experimental conditions and developing more reliable CNV calling algorithms.\n\n\n\nIn the meantime, it is required for individual researchers to know the characteristics of the available platforms and analytical techniques to use them or to interpret the published results properly.e found peak evidence of linkage (LOD score=1.88) for HDL cholesterol level on chromosome 6 (nearest marker D6S1660) and potential evidences for linkage on chromosomes 1, 12 and 19 with the LOD scores of 1.32, 1.44 and 1.14, respectively.\n\n\n\nThese results should pave the way for the discovery of the relevant genes by fine mapping and association analysis.IIntroduction.\n\n\n\nCholesterol is a major part of cell membranes.\n\n\n\nCholesterol is carried in the blood by chylomicrons, very low density lipoproteins (VLDL), high density lipoproteins (HDL) and low density lipoproteins (LDL) (Dastani et al.\n\n\n\n2006).\n\n\n\nHDL cholesterol is reversely associated with cardiovascular disease, and is more tightly controlled by genetic factors than the other lipoproteins such as LDL, VLDL and chylomicrons.\n\n\n\nEnvironmental factors including chronic alcoholism, estrogen replacement therapy, and exercise influence the levels of HDL cholesterol.\n\n\n\nSeveral families with strikingly elevated HDL cholesterol levels have been identified.\n\n\n\nHDL cholesterol levels are higher in blacks compared with whites and HDL cholesterol levels of females are higher than those of males (Barcat et al.\n\n\n\n2006; Brousseau et al.\n\n\n\n2004; Yamashita et al.\n\n\n\n2000; Imperatore et al.\n\n\n\n2000).\n\n\n\nCandidate gene analysis using population-based case-control studies has been used to test the association between SNPs and HDL cholesterol levels.\n\n\n\nAmong the candidate genes selected mainly from lipid metabolism pathways, ApoA-I gene is the one most intensively studied (Inazu et al.\n\n\n\n1994; Kuivenhoven et al.\n\n\n\n1997).\n\n\n\nBy genome-wide linkage analysis, susceptibility genes can be identified although the genes are not candidates based on lipid metabolism.\n\n\n\nGenome-wide linkage scans are conducted by use of microsatellite markers to identify genetic determinants affecting the traits (Wang and Paigen 2005).\n\n\n\nUsing HDL cholesterol levels as either discrete or quantitative trait, several linkage studies on genetic determinants of HDL cholesterol have been reported (Yancey et al.\n\n\n\n2003).\n\n\n\nGenetic effects on the variations in HDL cholesterol were studied mainly in Caucasians and Africans thus far, and little attention has been focused in this regard on Asian populations.\n\n\n\nWe found suggestive evidence for linkage for HDL cholesterol on chromosome 6, 1, 12 and 19, in studies conducted as part of GENDISCAN study, a large epidemiological study of Complex traits in geographically, culturally and genetically isolated large Mongolian families l in Dornod, Mongolia report.\n\n\n\nMethods.\n\n\n\nWe analyzed data from 1002 Mongolian individuals from 95 large extended families.\n\n\n\nInformed consent was obtained from all subjects prior to participation and the protocol was approved by the Institutional Review Board at Seoul National University.\n\n\n\nPotentially confounding variables were assessed for each participant along with overall medical history.\n\n\n\nInformation on age, gender and anthropometry (height, weight, waist circumference, hip circumference and body fat content) were obtained for each individual.\n\n\n\nHeight in centimeter (cm) and weight in kilograms (kg) were measured using an automatic measuring instrument (IMI 1000, Immanuel Elec., Korea).\n\n\n\nBody mass index (BMI) was calculated in kg/m.\n\n\n\nWaist circumference was measured to the nearest centimeter at the level of the umbilicus, and hip circumference was measured at the level of the maximal circumference of the gluteus.\n\n\n\nAll other variables were collected through interviews performed by trained interviewers.\n\n\n\nInformation about amount of alcohol and smoking was also obtained from all the participants.\n\n\n\nAll the subjects were asked to fast for 12 hours before their visit.\n\n\n\nBlood samples were collected from an antecubital vein into vacutainer tubes containing EDTA.\n\n\n\nBlood samples were centrifuged at 3000rpm for 10 minutes and then stored at 70C.\n\n\n\nDNA was isolated from lymphocytes for polymerase chain reaction (PCR) and automated genotyping.\n\n\n\nA 10 ml blood sample was collected from each participating individual for genomic DNA extraction.\n\n\n\nDNA was extracted from peripheral lymphocytes using the PUREGENE DNA Purification Kit for whole blood (Gentra Systems Inc, USA).\n\n\n\nFor genotyping, a set of 1000 microsatellite markers deCODE mapping sets (deCODE genetics, USA) was used covering the genome at an average density of 3 centimorgans (cM).\n\n\n\nHDL cholesterol was measured by the enzymatic method using Cholestest-N-HDL kit (DAICHI, JAPAN) and HITACHI 7600-210 \u0026 HITACHI 7180 instruments.\n\n\n\nExtensive quality control procedures ensured the validity and reproducibility of the measurements.\n\n\n\nMultiple linear regression analysis was used by PC SAS version 8.2 and PC SPSS version 12 to account for effect of confounding variables.\n\n\n\nPedigree data was managed by PedSys (Southwest Foundation for Biomedical Research, San Antonio, Texas, USA).\n\n\n\nNonpaternity was examined using PEDCHECK (Mcpeek and Sun 2000) and relationships other than paternity were checked using average IBD-based method by PREST.\n\n\n\nAfter correcting pedigree error and Mendelian errors, non-mendelian errors were examined and corrected using SimWalk.\n\n\n\nIdentity by descent (IBD) matrix between every relationship pairs in family was calculated and IBD matrix for single marker was calculated by SOLAR (Sequential Oligogenic Linkage Analysis Routines software version 2.1.4).\n\n\n\nMultipoint IBD matrices were computed on every 1 cM distance using Markov chain Monte Carlo method by LOKI (Heath 1997).\n\n\n\nGenetic components of selected phenotypes were estimated in terms of heritability.\n\n\n\nNarrow sense heritability, defined as the proportion of total phenotypic variation due to additive genetic effects, was calculated.\n\n\n\nHeritability of HDL cholesterol adjusted for age, gender, age- square, product of age and gender, product of age- square and gender, systolic BP, smoking and alcohol was estimated and a variance component linkage analysis was carried out by SOLAR which uses maximum likelihood methods to estimate variance components for the polygenic genetic effect and random individual environmental effects.\n\n\n\nResults and Discussion.\n\n\n\nThe mean age of the 1002 individuals was 31 years and 54.5% of them were female.\n\n\n\nDemographic and pedigree characteristics of the study sample are shown in Table 1.\n\n\n\nThe family size had a mean of 16.\n\n\n\nTable 2 included information on 2546 pairs of first degree relatives (1812 parent-offspring pairs and 734 full-sib pairs), 2485 pairs of their second degree relatives (395 half-sibling pairs, 1202 grandparent-grandchild pairs, and 888 avuncular pairs), and 598 first-cousin pairs.\n\n\n\nMeans of their total cholesterol, HDL cholesterol, LDL cholesterol, and triglyceride were 159.82 mg/dl, 55.19 mg/dl, 90.51 mg/dl, and 63.30 mg/dl, respectively.\n\n\n\nTable 3 shows correlation between HDL cholesterol and covariates such as age, gender, systolic blood pressure, alcohol consumption status, and smoking status.\n\n\n\nThese parameters were used as covariates in the variance component analysis which provided multivariable adjusted heritability estimates for HDL cholesterol of 0.45 (Table 4).\n\n\n\nThe peak multipoint LOD score was 1.88 on 6p21 (nearest marker D6S1660) and a secondary peak (LOD score of 1.44) was found on 12q23 (nearest marker D12S354).\n\n\n\nWe identified other potential evidence for linkage in the LOD score of 1.32 on 1q24 (nearest marker D1S412) and a LOD score of 1.14 at 19p13 (nearest marker D19S884) (Fig.\n\n\n\n1, 2).\n\n\n\nTable 5 presents all LOD scores 1.0 for HDL cholesterol.\n\n\n\nWe identified potential evidence of linkage on several chromosomes.\n\n\n\nIn other genome scan, a weak linkage signal for HDL cholesterol was observed for regions that overlapped slightly with the regions identified herein.\n\n\n\nKlos et al.\n\n\n\nreported the appearance of peak position in the chromosome 12q in European American population (Klos et al.\n\n\n\n2001) (Table 6).\n\n\n\nWe found evidence of link- the population isolates used in GENDISCAN study would not present significant inflation of type I errors from inbreeding effects in its gene discovery analysis.\n\n\n\nIIntroduction.\n\n\n\nThe GENDISCAN (Gene Discovery for Complex traits in Asian population of Northeast area) study was launched in 2002 in order to elucidate genetic causes of complex diseases.\n\n\n\nThis study attempted to incorporate designs that detect genetic signals with increased efficiency.\n\n\n\nThese included using genetically homogeneous population, recruiting large families, and considering quantitative phenotypes as well as disease outcome (Peltonen et al., 2001; Merikangas et al., 2003).\n\n\n\nLarge extended families still remaining in the Northeast Asia, enabled the project to adopt these designs.\n\n\n\nAlthough there is no doubt that gene discovery of common complex diseases is one of the research priorities, the successful results have been very limited (Grant et al., 2006).\n\n\n\nThe difficulty of replication across studies, mandates the use of internally valid study designs and proper methodologies.\n\n\n\nUsing population isolates generally confers the advantage of increasing genetic homogeneity.\n\n\n\nHowever population isolates might have inbreeding structures, which deviates the basic assumptions of HWE.\n\n\n\nThe presence of significant inbreeding necessitates modifications in genetic estimations using the population.\n\n\n\nTherefore, we attempted to estimate the status of HWE, and inbreeding coefficients in two ethnic groups of Mongolia using genome-wide short tandem repeat (STR) genetic markers.\n\n\n\nCompatibility with basic assumptions of population genetics can support the methodological validity of the overall GENDISCAN study,Methods.\n\n\n\nThe GENDISCAN study included non-selected families in Mongolia.\n\n\n\nThe People's Republic of Mongolia (not including the Chinese territory) has 2.6 million people which comprise of more than 20 ethnic groups.\n\n\n\nThe Orkhontuul are in Selenge Imag (Imag is an administrative district unit in Mongolia corresponding to a state in the United States) and the Dashbalbar area in Dornod Imag were selected.\n\n\n\nThe Orkhontuul area has a population of 3,760 people, mainly consisting of Khalkha tribe, and maintains semi-urban life style.\n\n\n\nThe Dashbalbar area is mainly habituated by about 4,000 people of Buryat ethnicity and has more traditional nomadic life style.\n\n\n\nMany large extended families, which fit the study purposes of the GENDISCAN study still remain in both areas.Genomic DNA was extracted from peripheral leukocytes.\n\n\n\nThe Orkhontuul samples (2004, n=1,080) were genotyped using the Applied Biosystems Inc. platform (ABI Prism Linkage Mapping Set version 2.5 medium density, 400 markers) with average 10 cM resolution, and Dashbalbar samples (2006, n=1,020) were genotyped using the deCODE 1,000 STR marker platform with average of 3 cM resolution.\n\n\n\nFor the Orkhontuul participants markers on the chromosome 14 were analyzed.\n\n\n\nFor Orkhontuul data, markers with low call-rate (49 markers), and with more than 1% of genotype error rates (16 markers) and markers on X chromosome (18 markers) were excluded.\n\n\n\nFor Dashbalbar genotype data, the 1,000 STR marker platform provided 1097 markers originally, however we excluded markers on X chromosome (49 markers) and markers with low call-rate and more than 1% of genotype error rates (4 markers).\n\n\n\nAll participants provided informed consent.HWE and degree of inbreeding were assessed using the founders of each pedigree.\n\n\n\nNon-founders were excluded because their genotypes are dependent on those of the founders.\n\n\n\nHWE was estimated by comparing the expected and observed genotype frequencies.\n\n\n\nExpected genotype frequency was calculated from allele frequency.\n\n\n\nChi-square goodness of fit test was used to determine whether HWE assumption was met.\n\n\n\nThe Chi-square statistics () of multi-allelic loci is defined as equation as Equation 1, with k (k-1) degree of freedom, where k is the total number of alleles.\n\n\n\n(Equation 1)where, nuu and nuv denote homozygotic and heterozygotic genotypes, while pu and pv denote allele frequency of each allele.\n\n\n\nInformation contents of the genetic markers were estimated as polymorphism information content (PIC), heterozygosity and allelic diversity.\n\n\n\nPIC is an index of the amount of information, which modifies the simple heterozygosity index by adjusting for the chance of mating between the same heterozygotic genotypes.\n\n\n\nPIC was calculated from Equation 2.\n\n\n\n(Equation 2)where p and p denote allele frequency of each allele (Czika, 2005).\n\n\n\nInbreeding was estimated by the deviation from the assumption that each founder shares no Identity by descent (IBD).\n\n\n\nGenerally genotype frequency of bi-allelic locus having p and q allele frequencies are predicted as p, 2pq, q respectively under HWE.\n\n\n\nHowever, if there are IBD sharing of FI between founders, above prediction can be re-written respectively as Equation 3.\n\n\n\n(Equation 3)where, Fdenotes inbreeding coefficient (Gillespie et al., 2004).\n\n\n\nIn brief, inbreeding is characterized by the excess of homozygote over expected level.\n\n\n\nThe inbreeding coefficient can be estimated as Equation 4 by solving Equation 3 (Equation 4)where, H denotes observed heterozygotic, and 2pq denotes estimated heterozygotic proportions from allele frequency (Hart et al., 2000).\n\n\n\nHWE and estimations of expected and observed heterozygosity frequencies were obtained using SAS/Genetics program.Results.\n\n\n\nThe demographic characteristics of the subjects geno-typed are shown in Table 1.\n\n\n\nThere were 280 (99 men and 181 women) and 142 (90 men and 52 women) founders in Orkhontuul and Dashbalbar populations.\n\n\n\nNon-founders' genotype.\n\n\n\nwere excluded, since theirs do not independently contribute to a gene pool.\n\n\n\nThe information contents in terms of PIC for single marker, range between 0.2 and 0.9, as shown in Fig.\n\n\n\n1.\n\n\n\nAverage PIC was 0.72 and 0.71 for Orkhontuul and Dashbalbar populations, respectively which are relatively high for single marker information contents.\n\n\n\nThere was no significant difference in PIC across the chromosomes or populations.\n\n\n\nThe high PIC level enabled accurate estimation of other population genetic parameters.\n\n\n\nHWE was satisfied among 88.6 % and 94.2%, respectively, of all markers in Orkhontuul and Dashbalbar populations (p-value 0.05).\n\n\n\nIf we apply the criteria of p-value 0.01, 90.5% and 95.3% of all markers were in HWE status All the markers including those which were not in HWE, were used for estimating the inbreeding coefficients,.\n\n\n\nInbreeding coefficient was estimated to be 0.0023 and 0.0021 in Orkhontuul and Dashbalbar populations.\n\n\n\nDiscussion.\n\n\n\nPopulation isolates are generally considered to be one of the most ideal populations for genetic study (Pajukanta et al., 2003; rcos-Burgos et al., 2002; Escamilla et al., 2001).\n\n\n\nHowever, possible inbreeding can cause deviation from general assumptions on which most analyses depend.\n\n\n\nPresence of inbreeding can be problematic, because, if exits, l the genetic relationships between unrelated as well as related persons could be underestimated.\n\n\n\nThis underestimation of IBD can result in inflation of type I errors for linkage analysis (Hossjer et al., 2006 Nomura et al., 2005), linkage disequilibrium estimations and haplotype reconstructions (Zhang et al., 2004).\n\n\n\nThe inbreeding coefficient found in this study (about 0.2% in each population), does not necessitate any adjustment for genetic analyses such as IBD calculation, classic or non-parametric linkage analysis, and variance component-based linkage analysis.\n\n\n\nBy estimating the last common ancestor, 0.2% of inbreeding coefficient corresponds to 10 or 11 generations (Jensen- Seaman et al., 2001; Santos-Lopes et al., 2007).\n\n\n\nIn this study, both ABI and deCODE STR markers were genotyped with standardized procedure and any markers with more than 1% of genotype errors were discarded.\n\n\n\nThe genotype errors were confirmed within the pedigree structure.\n\n\n\nAny Mendelian inconsistency was deleted and markers with possible double-recombination were also deleted.\n\n\n\nGenerally, genotyping in family-based study is more accurate than in studies using individuals only.\n\n\n\nThus, It is not likely that any genotype error could have been biased our findings.\n\n\n\nIn conclusion, we have estimated inbreeding coefficients in two population isolates in Mongolia.,.\n\n\n\nWe found that they fall in negligible range, allowing related genetic studies to be performed without any modification or adjustment for possible inbreeding effects.\n\n\n\nThis finding validates the ability of The GENDISCAN study to add to the growing body of evidence which associates specific genetic variations with complex disorders.% (6.4 of 34.5 Mb) of chromosome 22 with 757 tagSNPs and 815 haplotypes (frequency 5.0%).\n\n\n\nOf 3430 common SNPs genotyped in all five populations, 514 were monomorphic in Koreans.\n\n\n\nThe CHB + JPT samples have more than a 72% overlap with the monomorphic SNPs in Koreans, while the CEU + YRI samples have less than a 38% overlap.\n\n\n\nThe patterns of hot spots and LD blocks were dispersed throughout chromosome 22, with some common blocks among populations, highly concordant between the three Asian samples.\n\n\n\nAnalysis of the distribution of chimpanzee-derived allele frequency (DAF), a measure of genetic differentiation, Fst levels, and allele frequency difference (AFD) among Koreans and the HapMap samples showed a strong correlation between the Asians, while the CEU and YRI samples showed a very weak correlation with Korean samples.\n\n\n\nRelative distance as a quantitative measurement based upon DAF, Fst, and AFD indicated that all three Asian samples are very proximate, while CEU and YRI are significantly remote from the Asian samples.\n\n\n\nComparative genome-wide LD studies provide useful information on the association studies of complex diseases.\n\n\n\nIIntroduction.\n\n\n\nVast amounts of information on single nucleotide polymorphisms (SNPs) and progress in high-throughput genotyping technology have generated a great deal of interest in establishing genome-wide linkage disequilibrium (LD) maps for genetic studies of complex traits (Chakravarti 2001; The International HapMap Consortium 2003; Myers and Bottolo 2005).\n\n\n\nLD is known to occur in a block-like structure across the genome, with conserved haplotype blocks of tens to hundreds of kilobases punctuated by \"hot spots\" of recombination (Daly et al.\n\n\n\n2001).\n\n\n\nSince the concept of whole genome association studies using SNPs was introduced (Risch and Merikangas 1996), an optimal number of SNPs required for association studies has been center of extensive debate (Kruglyak 1999).\n\n\n\nInitial studies have focused on average LD levels and the variability in processes that generate LD (Cardon and Abecasis 2003).\n\n\n\nAlthough a single chromosome could carry many haplotypes in LD blocks, recent studies suggest that haplotypic variation may be much lower than previously imagined (Jeffreys et al.\n\n\n\n2001; Patil et al.\n\n\n\n2001; Gabriel et al.\n\n\n\n2002).\n\n\n\nPatil's group identified haplotype blocks on chromosome 21 for which over 80% of chromosomes were represented by a few common haplotypes (Patil et al.\n\n\n\n2001).\n\n\n\nIn the analysis of human chromosome 22 with a marker density of one SNP per 15 kb, Dawson's group reported a highly variable pattern of LD along the chromosome, in which extensive regions of complete LD of up to 804 kb in length were interspersed with regions of no detectable LD (Dawson et al.\n\n\n\n2002).\n\n\n\nAlthough differences of LD patterns between populations have been reported (Abecasis et al.\n\n\n\n2002; Reich et al.\n\n\n\n2001, Zavattari et al.\n\n\n\n2002), little information is available on the haplotype structure in different populations other than the recent study by S.B.\n\n\n\nGabriel, et al.\n\n\n\n(Gabriel et al.\n\n\n\n2002).\n\n\n\nOn the other hand, haplotype analysis has been widely employed in linkage studies for narrowing down the location of disease susceptibility genes (Zhang et al.\n\n\n\n2004; Park 2007).\n\n\n\nThe International HapMap Project was launched to develop a haplotype map of the human genome, the HapMap, which will describe the common patterns of human DNA sequence variation among four population samples: 30 trios from Yoruba in Ibadan, Nigeria (YRI), 45 unrelated Japanese in Tokyo, Japan (JPT), 45 unrelated Han Chinese in Beijing, China (CHB), and 30 trios in a Utah, US population with Northern and Western European ancestry (CEU) from the CEPH collection (The International HapMap Consortium 2003; 2004; 2007).\n\n\n\nAs the International HapMap Project releases a validated SNP map of 1 marker per kb for the HapMap samples, the general applicability of the HapMap data needs to be confirmed in samples from related populations.\n\n\n\nRecent comparative studies of LD patterns have shown a high degree of concordance among various populations (Gabriel et al.\n\n\n\n2002; Shifman et al.\n\n\n\n2003; Stenzel et al.\n\n\n\n2004; Mueller et al.\n\n\n\n2005).\n\n\n\nAs the HapMap samples include Japanese and Chinese, it was our interest to test whether significant differences in LD exist between Koreans and the two other Asian samples.\n\n\n\nIn this paper, we measured the LD pattern along chromosome 22 in Korean samples and compared the Korean data with those of the four HapMap samples.\n\n\n\nWe were interested in exploring how the HapMap data could be used to estimate the genomic structure of Koreans.\n\n\n\nWe expect that this study will contribute to the development of proper strategies for association studies of common complex diseases in Koreans using the HapMap data.\n\n\n\nMethods.\n\n\n\nA total of 111,448 reference SNPs from chromosome 22 in the dbSNP (http://www.ncbi.nlm.nih.gov/SNP, build 116) were collected.\n\n\n\nTo maximize cost effectiveness of genotyping, SNPs were selected based on the following criteria: 1) markers with even spacing, 2) verified SNPs, 3) coding SNPs.\n\n\n\nThe SNPs were scored for the selection of the study using the following strategies.\n\n\n\nFirst, it was most important in mapping chromosomal LD blocks to have relatively equal spaces between SNP markers.\n\n\n\nSecond, verified SNP markers (validation status was scored as 0 to 4 in the dbSNP) that had higher scores were chosen to prevent or reduce genotyping failure.\n\n\n\nAlso, repeated sequence regions were excluded by repeat masking with Primer3 software (Rozen and Skaletsky 2000).\n\n\n\nThird, to be useful for a further study, protein coding SNPs had higher scores.\n\n\n\nA total of 12,674 genotyping experiments were conducted by four Genotyping Centers, and a final set of 4681 markers passed the stringent quality control procedure (The International HapMap Consortium 2003).\n\n\n\nGenomic DNA from 90 unrelated Korean individuals without family histories of major diseases was obtained from the Genomic Research Center in the Korean National Institute of Health (KNIH).\n\n\n\nThe KNIH samples were collected as part of an epidemiological project and represent urban and rural regions in the south of Seoul.\n\n\n\nThe sex ratio was 0.5 and the mean age was 50.\n\n\n\nInformed consent from all participating subjects was obtained through KNIH, and research approval came from the relevant ethical committees.\n\n\n\nDNA was isolated from peripheral blood leukocytes according to standard procedures with proteinase K-RNase digestion, followed by phenol-chloroform extraction.For each SNP, we chose a set of three primers: two PCR primers to amplify a product of 100-200 bps under standard conditions and an optimized extension primer to be complementary to the sequence immediately to a SNP site.\n\n\n\nFor genotyping, we employed three platforms-6063 SNP genotypings were done using the Orchid Bioscience SNP-IT assay (Princeton, NJ), 984 SNP genotypings using the PerkinElmer Life Sciences FP-TDI assay (Boston, MA), and 5627 SNP genotypings using the Sequenom MassARRAY (San Diego, CA).\n\n\n\nA genotype frequency for each SNP was checked for consistency between the observed values and those expected from the Hardy-Weinberg equilibrium test in each assay.\n\n\n\nHaploview version 3.2 (Barrett et al.\n\n\n\n2004), based on the expectation-maximization (EM) method (Excoffier and Slatkin 1995), was used to infer haplotype phase and population frequency and to estimate the Lewontin's coefficients D' (Lewontin 1998), LOD, and correlation coefficient r (Hill and Robertson 1968).\n\n\n\nPHASE v2.1 was used to estimate the recombination parameters (Li and Stephens 2003; Crawford et al.\n\n\n\n2004) and assess the statistical significance of haplotype profile differences and individual haplotype fre-2006).\n\n\n\nBecause it has been suggested that the functional significance of IL-1B-3737 might depend on a broader haplotype, we used the three SNPs for haplotype analysis.\n\n\n\nHaplotypes were reconstructed by PHASE version 2.1, using previously produced genotype data (Lee et al., 2004).\n\n\n\nOf the possible eight haplotypes, three common ones accounted for 98% of the estimated haplotypes in the Korean population.\n\n\n\nTable 1 shows the haplotype frequency estimation in each population.\n\n\n\nThe potentially more inflammatory IL-1B-511T/-31C haplotype represented 53.5% of the Korean haplotypes, compared with 33.7% of the Caucasian haplotypes.\n\n\n\nSo far, in many previous association studies, the individual SNP approach, most frequently using IL-1B-511 and IL-1B-31, has been adopted.\n\n\n\nTo our knowledge, we reported first that the IL-1B-1464 polymorphism has allele-specific differences in nuclear protein binding and is associated with a clinical disease (Lee et al., 2004).\n\n\n\nThe biological implication of this polymorphism was supported by in vivo studies by Chen et al.\n\n\n\nthat showed that the IL-1B-1464 polymorphism has substantial allele-specific effects when both IL-1B-511 and IL-1B-31 were alleles T and C, respectively (Chen et al., 2006).\n\n\n\nThe more informative haplotype 1 (GTC), containing the IL-1B-1464 polymorphism, which shows the highest transcriptional activity, represents 9.3% and 6.0% of Korean and Caucasian haplotypes, respectively, whereas haplotype 3 (GCT), with the lowest activity, had a higher frequency in Caucasians (64.8%) when compared with Koreans (44.2%) (Table 1).\n\n\n\nThe difference in IL-1B promoter haplotype frequency between the Korean and Caucasian populations was statistically significant (=20.6, p=0.000), and the allele frequencies of the IL-1B-1464 polymorphism (rs#1143623) were also significantly different between the two populations (IL-1B-1464 G allele frequencies for Korean and Hapmap European=0.548 and 0.672, respectively) (=6.38, p=0.01).\n\n\n\nIt has been suggested that genes that are involved in immune function may be under selective pressure in direct interaction with the environment (Sawyer et al., 2004; Kim et al., 2005).\n\n\n\nThe genes that influence a phenotypic variation between populations are expected to show high Fst values.\n\n\n\nCompared with the Fst value for the Caucasian-vs-Asian comparison, the Fst values for the African-vs-Asian or -Caucasian comparisons were remarkably high (Fig.\n\n\n\n1).\n\n\n\nPreviously, we reported that the IL-1B-1464 polymorphism contributes to the development of intestinal-type gastric cancer among Koreans (Lee et al., 2004).\n\n\n\nAs a curious finding in our report, the editor pointed out that carriers of IL-1B-1464 G tend to have a decreased risk of diffuse-type of gastric cancer, which is the opposite of intestinal-type gastric cancer, although both intestinal and diffuse types of gastric cancer are related to Helicobacter pylori-induced gastritis (Furuta et al., 2004).\n\n\n\nOur results showed that most IL-1B-1464 C alleles are linked to the IL-1B-511T/-31C haplotype (Table 1).\n\n\n\nConsidering the level of promoter activity of haplotype 2 (CTC), we cannot exclude the possible association between this haplotype and the risk of diffuse-type gastric cancer, especially depending on interactions with other regulatory factors (Lee et al., 2007).\n\n\n\nAssociation studies that use individual SNPs appear to be insufficient, and the understanding of functional haplotype structure of populations could provide potential explanations for IL-1B-related controversies and ethnic-specific associations.\n\n\n\nTherefore, we believe that these Korean haplotype data will be useful for future association studies between IL-1B SNPs and disease risk.nted domains, including the human imprinted gene cluster that contains IGF2, H19, KCNQ1, ASCL2, and CDKN1C (Rapkins et al., 2006).\n\n\n\nIf, as has been suggested, imprinted genes are intimately connected with the acquisition of parental resources, we would not anticipate the existence of such genes in chicken, which leave their offspring to their own heritance after conception.\n\n\n\nPhylogenetic analyses expose that the relationship between human and mouse is closer than that between human, mouse, and chicken.\n\n\n\nSimilarly, the relationship between zebrafish and chicken is quite distant (Shah et al., 2004).\n\n\n\nNonetheless, we assumed that chicken have imprinted genes due to the existence of common ancestral genomic regions that have evolved on a similar basis in each of the aforementioned species.\n\n\n\nThe purpose of this study was to identify candidate imprinted genes in chicken based on an analysis of orthologous genes in human, mouse, zebrafish, and chicken using the HomoloGene database.ols for the clinical oncology to determine the prognosis of patients (Lossos et al., 2004; Pomeroy et al., 2002), the molecular diagnosis (Golub et al., 1999) as well as the responsiveness to therapeutics (Snyder and Morgan, 2004).\n\n\n\nThere have been many reports on the molecular pattern analysis using microarray to understand the chemo- and radio-resistance in cervical cancer (Achary et al., 2000; Tewari et al., 2005; Wong et al., 2006), rectal cancer (Kim et al., 2007) and esophageal cancer (Fukuda et al., 2004).\n\n\n\nMost of the studies are to identify differentially expressed genes in patients with different clinical outcomes, which can be applied to the evaluation of prognosis more accurately.\n\n\n\nAlthough the conventional parameters like tumor stage and grade can be used to decide optimal cancer therapy, molecular markers would provide valuable information to make clinical decisions (Klopp and Eifel, 2006).\n\n\n\nGenome-wide analysis on gene expression can predict the clinical consequences more accurately.\n\n\n\nIn addition, the information from gene expression profiling can facilitate the development of biological target for therapeutics by identifying pathways and determining steps contributing to the phenotype.\n\n\n\nIn this study, we examined the expression profiles of two lung cancer cell lines, which showed differential re- 1995).\n\n\n\nIn the inactive form, the pseudosubstrate domain is bound to the catalytic domain of PKC (Orr et al, 1994).\n\n\n\nUpon stimulation, PKC translocates to the plasma membrane where the C1 and C2 domains interact with DAG and phosphatidylserine, respectively.\n\n\n\nThis interaction causes the pseudosubstrate domain to dissociate from the catalytic domain, which results in activation of PKC.\n\n\n\nInactive PKC is not freely distributed throughout the cytoplasm but appears to be localized to specific sites within the cell.\n\n\n\nAssociation of PKC with scaffolding proteins such as AKAP79 (A Kinase-Anchoring Protein 79) (Klauck et al, 1996) and Gravin (Nauert et al, 1996) facilitates localization.\n\n\n\nStreptomycetes are ubiquitous soil bacteria, and they play a key role in the global carbon cycle by degrading the insoluble remains of other organisms.\n\n\n\nMore clues to the development of the PKC super family come from the study of the bacterium Streptomyces coelicolor.\n\n\n\nS. coelicolor has a large collection of enzymes and can metabolize many diverse nutrients.\n\n\n\nThis extremely simple organism contains approximately 8,667,507bp, yet has complex life cycle exhibiting mycelial growth and spore formation (Bentley, 2002) and notable for production of pharmaceutically useful anti-tumor compounds.\n\n\n\nOf the predicted genes, an unprecedented proportion carries out regulatory functions in the cell (Winstead, 2002).\n\n\n\nMore than twelve percent of the genome is involved in facilitating biological processes, such as the bacterium's s reduce implementation time and increase the likelihood of eliminating bugs and localizing code modifications when a change in implementation is required.\n\n\n\nIn the initial version of the interface, all of the classes got tangled with each other and corrupted the concept of object-oriented programming.\n\n\n\nHowever, they have been completely redesigned, as shown in Table 1.\n\n\n\nThis table summarizes the recent modifications of our system, and the interfaces for each class are documented, similar to Fig.\n\n\n\n2.\n\n\n\nThe refactored version is now composed of 3851 lines, compared with the initial version, which was composed of 2765 lines of code.\n\n\n\nBy importing the five packages, an exemplary software system called J3dPSV 1.0, shown in Fig.\n\n\n\n3, has been developed for viewing 3D structures of proteins from the Protein Data Bank for demonstrational purposes.\n\n\n\nJ3dPSV supports visualization of proteins for educational purposes by simulating simple molecular graphics.\n\n\n\nIn addition, J3dPSV interactively displays a molecule on the screen in a variety of color schemes, molecular representations, and animation features.\n\n\n\nThe molecular model can be changed by selecting the list (cartoon tubes, backbone, protein, cylinder, or line) in useful suggestions for genotype information.\n\n\n\nCompared to the current genotyping tools, GTVseq has several unique and useful features in the following aspects: * GTVseq uses two different scoring schemes and the results are reported separately.\n\n\n\nOne of the scoring schemes is similar to that of NCBI, while the other is particularly useful for viral sequences with new or complicated genotypes (vide infra).\n\n\n\n* GTVseq offers an easy and interactive web-based user interface, with intuitive reports for genotyping results.\n\n\n\n* GTVseq can be used for genotyping many important viruses such as HIV-1, HIV-2, HBV, HCV, HTLV-1, HTLV-2, poliovirus, enterovirus, flavivirus, Hantavirus, and rotavirus, thus permitting the most comprehensive genotyping of viral genomes to date.Methods.\n\n\n\nFor genotyping of viral genome sequences, we need to establish 'reference sequences' for each genotype.\n\n\n\nWe have downloaded the reference sequence database collections from NCBI (http://www.ncbi.nlm.nih.gov/projects/ genotyping), for HIV-1, HIV-2, HBV, HCV, HTLV-1, HTLV-2, and poliovirus.\n\n\n\nFor HIV-1 reference sequences, GTVseq also provides several different collections of reference databases such as HIV-1 (2004) \u0026 CRF, HIV-1 (2005), HIV-1 (2005) \u0026 CRF.\n\n\n\nFor enterovirus, flavivirus, Hantavirus, and rotavirus, the reference sequences were combination of databases and interactive web pages for manipulating and displaying annotations on genomes.\n\n\n\nIn other words, GBrowse is a web-based application tool that is developed for navigating and visualizing the genomic features and annotations interactively for users.\n\n\n\nThrough it, users can view a certain region of the desired genomes and search for genetic biomarkers.\n\n\n\nThey may conduct a full-text search for most features of the genomes.\n\n\n\nThey also can download SNP assay, genotype, and allele frequency information and generate customized sets of tag-SNPs for their association studies (Thorisson et al., 2005).\n\n\n\nGBrowse utilizes a web-based display that can be used to show arbitrary features of a nucleotide or protein sequence and can accommodate genome-scale sequences that are megabases in length.\n\n\n\nThe GBrowse system consists of various kinds of software modules and systems, such as web servers, database systems, and Perl libraries.\n\n\n\nAt present, many biological websites that provide genomic variants or portal services have been developed using GBrowse, including the following: the UCSC Genome Browser (Kuhn et al., 2007), the International HapMap Project (Thorisson et al., 2005), PlasmoDB (The is database is free for non-commercial purposes.\n\n\n\nThe KRDD is visualized using a web-based graphical view, and anonymous users can query and browse the data using the search function.\n\n\n\nThe KRDD homepage is shown in Fig.\n\n\n\n1, and the stored data are visualized using a web-based graphical view.\n\n\n\nIt has four major menus of web pages: (i) a Blast Search of a mutant line; Blast from rice Ds-tagging mutant lines; (ii) a primer design tool to identify genotypes of Ds insertion lines; (iii) a Phenotype menu for Ds lines, searching by gene name and phenotype characteristics among specific Ds lines; and (iv) a Management menu for Ds lines.\n\n\n\nThe Blast Search is searchable by selecting specific databases, consisting of DS Sequence, Indica Core, Japonica Core, Indica EST, Japonica EST, Indica Genome, Japonica Genome, Indica GSS, and Japonica GSS in Oryza sativa.\n\n\n\nThe KRDD uses several reference databases to facilitate a comprehensive analysis of the genome sequence.\n\n\n\nThese include the Entrez nucleotide database of the National Center for Biotechnology entative biological pathway database, now provides the KEGG Metabolism Atlas (Okuda et al., 2008) by manually combining about 120 existing metabolic pathway maps, as shown in Fig.\n\n\n\n1.\n\n\n\nHowever, the static approach to representing metabolic pathway diagrams offers no flexibility.\n\n\n\nOn the other hand, our initial attempts to visualize all information automatically in a single atlas map resulted in a confusing diagram that was difficult to interpret, as shown in Fig.\n\n\n\n2.\n\n\n\nIt should be noted that Fig.\n\n\n\n2 differs in many aspects from Fig.\n\n\n\n1 or conventional drawings in biochemistry textbooks.\n\n\n\nFor this reason, we designed a new metabolic atlas viewing tool called J2dpathway, which has node-abstracting features.\n\n\n\nWhen J2dpathway is initially executed, a window frame appears, as shown in Fig.\n\n\n\n3.\n\n\n\nThe screen consists of views and editors.\n\n\n\nThe tool-bar menu at the top lists various tool icons, including zoom-in, zoom-out, cliques, highly connected nodes, and obtaining cycles.\n\n\n\nThe Map Repository View on the left side lists a preinstalled data source that has many example pathways to explore, which are arranged in a tree view of the components of of the HIF1ODD domain with multiple partner proteins, such as ARD1 (Jeong et al., 2002), prolyl hydroxylase (PHD) (Schofield and Ratcliffe, 2004), and p53 (Fels and Koumenis, 2005; Sanchez-Puig et al., 2005), also have been reported.\n\n\n\nHowever, the molecular basis for the multiple binding specificity of the HIF1ODD domain has not been understood yet.\n\n\n\nThe detailed characterization of the correlation between the binding sequence motifs in the ODD domain and its binding to multiple target proteins is necessary for understanding the versatile function of the HIF1ODD domain.\n\n\n\nTwo functionally independent sequence motifs, the N-terminal and C-terminal ODD (NODD and CODD), in the HIF1ODD domain were shown to bind to the DNA-binding domain (DBD) of p53 (Hansson et al., 2002).\n\n\n\nThe crystal structure of the CODD motif in complex with pVHL was determined to ncluding 1p36, 5q31, and 21q22, by whole-genome linkage analysis (genome-wide association studies), and many polymorphisms also have been identified at these loci (Suzuki et al., 2003; Tokuhiro et al., 2003).\n\n\n\nHuman leucine-rich alpha-2-glycoprotein 1 (LRG1) was first identified as a trace protein in human serum (Haupt \u0026 Baudner, 1977).\n\n\n\nThe LRG1 gene is located on chromosome 19p13.3, and the primary sequence of LRG includes repeated leucine residues and also has putative membrane-binding domains.\n\n\n\nSerum LRG1 is the first extracellular ligand for cytochrome c (Cyt c).\n\n\n\nCyt c is a ubiquitous, heme-containing protein that normally resides in the space between the inner and outer mitochondrial membranes (Newmeyer et al., 2003).\n\n\n\nExtracellular Cyt c may play a role in inflammation, as it has been reported to cause arthritis when it is injected into mice.\n\n\n\nIts levels in RA patients' sera are significantly lower than those of healthy controls (Pullerits et al., 2005).\n\n\n\nAt least eight repeating 24-amino acid segments that have a notable consensus sequence were identified in a large family of LRG proteins.\n\n\n\nThe function of LRG has not been elucidated, although the functions of many of the other members of the LRR (leucine-rich repeat)-containing superfamily are known (Kobe \u0026 Deisenhofer, 1994; Buchanan \u0026 Gay, 1996).\n\n\n\nPlasma LRG expression levels are lower in liver cancer patients who are treated with radiofrequency ablation (Kawakami eutic agent for cancer is the in vivo specificity of cancer cell regression.\n\n\n\nFor such a specificity, target RNA-independent and nonspecific transgene induction by the group I intron should be avoided.\n\n\n\nIn other words, mis-spliced products should not be generated by the group I intron.\n\n\n\nIn this study, in order to evaluate the therapeutic feasibility of the hTERT-specific group I intron, we assessed the target RNA specificity of the trans-splicing phenomenon by the intron in mice that have been intraperitoneally xenografted with human cancer cells.Construction of Adenoviral Vector for hTERT-specific Group I Intron .\n\n\n\nThe expression vector that encodes for the hTERT-specific trans-splicing group I intron was constructed as previously described (Kwon et al., 2005; Song et al., 2006).\n\n\n\nIn brief, the Rib21AS group I intron, which recognizes uridine at position 21 (U21) of hTERT RNA, was generated to harbor an extended internal guide sequence, which includes an internal guide sequence (IGS, 5'-GGCAGG-3'), an extension of the P1 helix, an additional 6-nt-long P10 helix, and a 325-nt-long antisense sequence that is complementary to the downstream region (30 to 354 residues) of the targeted U21 of hTERT RNA.\n\n\n\nIn addition, cDNA, as a 3' exon that encodes for the lacZ gene, was inserted downstream of the modified group I intron expression construct (Fig.\n\n\n\n1A).\n\n\n\nusing PHRAP (http:// www.phrap.org/), it does not ensure correct assembly because the quality scores that are generated from 454 data are not compatible with those from Sanger reads.\n\n\n\nFurther, PHRAP has problems with handling massive reads (usually hundreds of thousands from an SFF file).\n\n\n\nA recent report has demonstrated that GS assembler programs (gsAssembler for de novo assembly and gsMapping for reference-guided assembly; http://www.\n\n\n\n454.com/enabling-technology/the-software.asp) that are supplied by Roche Applied Science are ideal for correct assembly of 454 data that are short and inherently error-rich (Chaisson and Pevzner, 2008).\n\n\n\nRecent versions (1.1.02.15 and later) of GS assembler programs support mixed assembly with Sanger-type reads, but their performance is not well known at present.\n\n\n\nMoreover, because pre-existing assembly software such as PHRAP and CelAsm (Huson et al., 2001) do not directly support data that are produced by 454 machines, 454-derived contigs (GS contigs) should be used as if they were individual reads or be shredded to generate many overlapping 'pseudoreads' (Goldberg et al., 2006).\n\n\n\nPseudoreads, made from GS contigs to emulate the read size of standard Sanger data (ca.\n\n\n\n600 bp), are virtual reads whose stepping between consecutive dertaken as a collaboration between Korean funding agencies (Ministry of Education, Science and Technology and Korean National Institute of Health), experimental academia (Ulsan Medical Institute, SungKyunKwan Medical Institute, and Korea Advanced Institute of Science and Technology), and corporations (DNA Link, SNP-Genetics, and Samsung Advanced Institute of Technology) (Yoo et al., 2006; Lee et al., 2008).\n\n\n\nResulting from the project, a Korean SNP and haplotype database system was developed to help those researchers who study high-frequency, complex Korean diseases and changes in ethnic global migratory variants.\n\n\n\nIn the project, we tried to accomplish a number of goals.\n\n\n\nFirst, the system should be able to provide essential information that is needed for gene discovery of complex Korean diseases.\n\n\n\nSecond, the system should contain basic and advanced tools that may apply to applications such as diagnostics, treatment, and prevention of diseases.\n\n\n\nThird, the database system should provide Korean-specific SNPs and haplotype information that are common in the Korean population.\n\n\n\nWe have developed a series of software programs for association studies as well as for the comparison and analysis of Korean HapMap data with four other populations (Yorubans in Ibadan, Nigeria; Centre d'Etude du are involved in lipogenesis, such as SREBF1, suggesting that PTP1B may play a role in the enlargement of adipocyte energy storage (Rondinone et al., 2002).\n\n\n\nThe human PTPN1 gene maps to chromosome 20q13.13, a syntenic region of the distal arm of mouse chromosome 2 that harbors quantitative trait loci for body fat and body weight (Lembertas et al., 1997).\n\n\n\nThe PTPB1 gene consists of 10 exons, spanning 74 kb, and the first intron is longer than 50 kb.\n\n\n\nIn humans, several linkage signals with type 2 diabetes mellitus (T2DM) (Bowden et al., 1997), BMI (Hunt et al., 2001), fat mass, and energetic intake (Collaku et al., 2004; Dong et al., 2003; Lembertas et al., 1997) were reported at this locus in different populations, further supporting the candidacy of PTPN1 involvement in T2DM and obesity.\n\n\n\nIn Poland, a family-based linkage study of T2DM showed the highest logarithm of the odds score (Ji et al., 1997; Klupa et al., 2000).\n\n\n\nThis locus also showed evidence of linkage with early onset T2DM (onset=45 years) in a subset of 55 French families (Zouali et al., 1997).\n\n\n\nprostaglandin and is associated with biologic events such as injury, inflammation, and proliferation (Hla and Neilson, 1992; Tazawa et al., 1994).\n\n\n\nPTGS2-mediated prostanoids play an important role in maintaining blood pressure (Anderson et al., 1976; Daniels et al., 1967).\n\n\n\nSpecially the cortical PTGS2- derived prostaglandin I2 participates in the pathogenesis of renal vascular hypertension through stimulating renal rennin synthesis and release (Hao and Breyer, 2008).\n\n\n\nClinical studies as well as animal studies also demonstrate important roles for PTGS2 in maintaining cardiovascular homeostasis (Zewde and Mattson, 2004; Zhang et al., 2006).\n\n\n\nPTGS2 is upregulated in animal models of cardiac failure (Abassi et al., 2001; Adderley and Fitzgerald, 1999), and its expression has been detected in heart failure in humans (Wong et al., 1998).\n\n\n\nPTGS2 gene is located on chromosome 1q25.2-q25.3 (Hla and Neilson, 1992) and its cDNA encodes a 604 amino acid protein.\n\n\n\nRecently a large-scale association study in Japanese population revealed the association of PTGS2 poly-c blood pressures and heart rate (Eric Colman, 2005).\n\n\n\nPhendimetrazine has also been widely prescribed as an anorectic for the treatment of obesity, and has been reported to have properties similar to methamphetamine, which is known to suppress appetite by activating catecholaminergic neurotransmission (Seiden et al., 1993; Chen et al., 2001).\n\n\n\nMethamphetamine is known to primarily block dopamine transporter, which inhibits dopamine reuptake, indicating that dopamine up-regulation has an anorectic effect (Mackler et al., 1993).\n\n\n\nBecause phendimetrazine and methamphetamine stimulate the central nervous system to produce euphoria, probably via the activation of dopaminergic systems in the brain (Nailles et al., 2003), these drugs are restricted to short-term use (a few weeks) and prominently labeled to warn against the risk of addiction.\n\n\n\nHowever, although many anorectics are available, evidence is still lacking concerning their efficacies, safeties, and molecular mechanisms.\n\n\n\nRecently, cDNA microarray studies on gene expression profile changes by amphetamine have been reported (Noailles et al., 2003; Yamamoto et al., 2005), but no such report has been issued on other anorectics.\n\n\n\nIn this study, we employed gned for the identification and visual representation of CNAs using genome-wide array-CGH profiles.\n\n\n\nCNAs can be directly identified from log2 ratio profiles that can be obtained from array-CGH datasets with minimal modifications.\n\n\n\nData smoothing option is also provided to cope with the noise level of data for reliable detection of CNAs.\n\n\n\nThe identification of CNAs is based on SW- ARRAY algorithm that ensures fast and robust detection of chromosomal alterations.\n\n\n\nThe identified CNAs are exported into Excel-compatible outputs or graphically illustrated with graphic-user interface.\n\n\n\nRelatively easy operability as well as the fast processing of overall procedures is the major advantage of our software over the conventional ones.\n\n\n\nCGHscape software package is freely available and provides the comprehensive environments for investigation of tumor genome and genomic variants.Major Functionalities of CGHscape.\n\n\n\n(1) CGHscape was designed as a standalone program compatible in Microsoft Windows environments.\n\n\n\nCompiled codes of CGHscape can be easily installed.\n\n\n\nThe interpreter- or web-based methods have the advantage al., 2006).\n\n\n\nGenealogical relationships among haplotypes in a chromosome 2 8.4 kb region without obligate recombination events were demonstrated using the CEU samples only (The International HapMap Consortium, 2005).\n\n\n\nIf other population samples such as YRI, CHB, and JPT had been included, the haplotype blocks would have been fragmented due to a number of historical recombination events and phylogenetic studies with such a small block would have not been informative.\n\n\n\nIn this study, instead of conventional tree-based phylogeny, principal coordinate analysis (PCoA) (Higgins, 1992) was employed using the haplotype data on a region encompassing multiple blocks.\n\n\n\nAs PCoA, albeit distance-based, is useful to grasp the major trend among the sequences, it would be worth to try how PCoA performs with such a dataset.\n\n\n\nAs an illustrative purpose, a region of 200 kb in chromosome Xq28, which is about 1 Mb away from the pseudoautosomal region (PAR2) at the tip of X chromosome long arm, was chosen and the haplotype structures of three ethnic groups that showed apparent recombination events were compared.\n\n\n\nThis region of the human genome harbors several important disease genes such as glucose-6-phosphate dehydrogenase (G6PD), cancer/testis antigens (CTAG1B, CTAG2), and Gab3 protein (GAB3).\n\n\n\noaches should help identify biomarkers to classify specific diseases based on high-throughput data.\n\n\n\nHowever, when a patient’s sample is evaluated to determine his/her disease status using more than one experimental condition relative to a determined biomarker set, correct prediction becomes impossible.\n\n\n\nFurthermore, methods to predict the disease status of a patient using biomarkers that initially are identified under different conditions than those that are used for the patient analysis have not been developed.\n\n\n\nThis study suggests a method that can accurately predict the disease status of a patient using a predetermined biomarker that is developed on a different platform.\n\n\n\nSpecifically, we performed a two-step discretization of gene expression values by their rank, which were processed in both the biomarker selection and prediction stages.Methods.\n\n\n\nTo evaluate our proposed method, we used two different datasets: the NCI dataset (Lee, et al., 2003) and the colon cancer dataset (Kim, et al., 2007; Notterman, et al., 2001).\n\n\n\nBoth of these datasets include gene expression information that was determined experimentally using two different microarray platforms (oligonucleotide-based and cDNA-based).\n\n\n\nThere are a large number a loss of function of the oxidase (Tosha et al., 2004).\n\n\n\nRecently, the I47A/I54V protease mutant in complex with Lopinavir showed that mutation affects the strain of the bound inhibitor in the protease-binding cleft (Grantz Saskova et al., 2008).\n\n\n\nIn previous studies, the mutation of specific sites has been shown to have an effect on the function and structure of proteins that cause disease.\n\n\n\nIt is well known that there is a correlation between mutated proteins and disease.\n\n\n\nAlso, there are bioinformatic tools to predict the correlation between mutation and disease, such as SIFT (Steven Henikoff et al., 2003) and PolyPhen (Vasily Ramensky et al., 2002).\n\n\n\nHowever, these tools are based only on sequence homology.\n\n\n\nIn this study, we conducted a large-scale structural and sequence mutational analysis of amino acids that could have a direct effect on protein function.\n\n\n\nBecause we collected the largest number of 3D structural changes in proteins, such as pockets, we named the dataset the structural mutatome.\n\n\n\nThe number of such structural mutations will increase continuously, and mapping the mutations to function and to disease will play a critical role in understanding the precise disease mechanisms that are caused by 3D mutations.\n\n\n\nWe classified mutated proteins by their structural properties (distance of pocket residue and mutation, pocket size, surface size, and stability) and physico-chemical properties (weight, instability, isoelectric point, and GRAVY cations that are related to the comparison and translation of various XML languages and parsers (Funahashi et al., 2004; Strmbck et al., 2005; Choi et al., 2008).\n\n\n\nHowever, because they were mostly aimed at drawing only relatively small-scale drawings that unify?only several pathways, systematic analyses of shared and duplicated compounds between pathway maps were not necessary.\n\n\n\nThus, to the best of our knowledge, KGML analyses tools rarely have been addressed in the literature to draw a large-scale pathway, such as the KEGG Atlas from a graph-theoretical perspective.\n\n\n\nAs a preliminary step in providing automatic graph layout techniques to the genome-scale flow of metabolism, analyzing KEGG XML files is crucial for software developers.\n\n\n\nThus, in this paper, we provide shared and duplicate compound information, using our XML analyses tool, to provide valuable information for automatic layout research in the area of systems biology.\n\n\n\nThese kinds of analyses that are based on graph-theoretical perspectives can be extremely useful when drawing a global pathway map in which edge crossing arises as a crucial issue.\n\n\n\nulting in a vast amount of genetic and pathway information with regard to the etiology of cerebrovascular disease.\n\n\n\nThese genes were annotated to access information on transcription, translation, structural function, and relatedness to the disease.\n\n\n\nIn addition to in silico data mining, 320 250K Affymetrix SNP chips (GeneChip Human Mapping 250K Nsp Array, Affymetrix, Inc., CA) were utilized for a case/control association study to generate experimentally associated markers of cerebrovascular disease.\n\n\n\nThe associated genes from the SNP chips and the genes that were retrieved from in silico data mining systems were compared and analyzed.\n\n\n\nA protein-protein network diagram that showed the integrated markers and their relationships was constructed in order to analyze the network characteristics and produce hub genes.\n\n\n\nIt was found that the PPI network that was associated with cerebrovascular disease follows a power-law degree distribution, as other biological networks do (Peri et al., 2003).\n\n\n\nThe PathwayStudio 5.0 program (Ariadne, Inc., MD, USA) was utilized to process the natural text mining of PubMed abstracts; the use of PathwayStudio resulted in a gene-disease association network.\n\n\n\nThe etiology of the disease and its related genes, which were extracted from in silico data mining and network analy-Transitional DTD standard and does not use technologies that are dependent on specific web browsers.\n\n\n\nThis is one way to make a web alignment tool more compatible with many web browsers.\n\n\n\nWe have developed a user-friendly a web based alignment tool based on ClustalW-MPI program.\n\n\n\nIt is standard and easy to maintain.\n\n\n\nThis web tool will help researchers to carry out multiple sequence alignment with a large number of input accompanies by a viewer and an editing function.\n\n\n\nIt also enables users to download the results and do basic analyses such as building trees and sequence clustering.Features and Results.\n\n\n\nIn order to use alignment tools, most advanced users use UNIX or Linux commands and options directly in a console window.\n\n\n\nIt is very inconvenient to use.\n\n\n\nIt also can cause frequent mistakes.\n\n\n\nA web alignment tool can be executed through a GUI environment on the web page by selecting commands and options.\n\n\n\nOur web alignment tool has the following features; input, downloadable output, and visualization.\n\n\n\nUsers input multiple sequences in the web alignment ty.\n\n\n\nThe speed of sequencing is advancing many folds per year, much faster than the cycle of semiconductor chips in computer industries.\n\n\n\nAlso, genome sequencing technology is becoming an everyday technology at the level as computer CPUs are universally used.\n\n\n\nIn five years time, experts predict that everyone in developed nations will be able to have his or her own genome information.\n\n\n\nDue to its far reaching consequences in medicine, health, biology, nanotechnology, and information technology, DNA sequencing will become the most important industrial technology ever developed during the next decades.Personal Genomics.\n\n\n\nIn 2009, genome sequencing technologies will achieve one person's whole genome per day in terms of DNA fragments sequenced.\n\n\n\nPersonal genomics is a new term that utilizes such fast sequencers.\n\n\n\nIn 2008, the cost for one personal genome is less than $350,000 USD.\n\n\n\nIf the cost goes down below $1,000 USD, the impact of personal genomics is predicted to be the largest ever in biology in common people's lives.\n\n\n\nReflecting this technological advancement to society is the PGP (Personal Genome Project), a project to sequence as many people as possible with lowest possible cost (Church, 2005).\n\n\n\nAt ited human diseases.\n\n\n\nIn addition, many computational programs have been created to predict the functional effects of unknown CVs (Ng et al., 2006; Care et al., 2007).\n\n\n\nDatabase searches and bioinformatic predictions can be useful in prioritizing novel CVs for further analysis.\n\n\n\nIn this review, we summarize the databases that are most helpful in interpreting the functional effects of CVs.\n\n\n\nWe perform an extensive survey of existing in silico prediction methods and compare their performance.\n\n\n\nFinally, we introduce a combination method as a promising approach to improve prediction performance.Polymorphism and Mutation Databases.\n\n\n\nSeveral databases that are helpful in assessing the functional effects of CVs or their relevance to disease phenotype are listed in Table 1.\n\n\n\nEach of two broad-category mutation databases, general mutation databases (GMDBs) and locus-specific mutation databases (LSDBs), has unique strengths and weaknesses (Porter et al., 2000).\n\n\n\nBecause polymorphism and mutation databases have been developed for different uses, they complement each other.d to the successful identification of specific positively selected genes, including human olfactory genes and human leukocyte antigen (HLA) loci (Salamon et al., 1999; Gilad et al., 2000).\n\n\n\nTherefore, the NS/S ratio test is a recognized tool for the effective detection of types of natural selection in protein-coding genes.\n\n\n\nUnder conditions of no selection, we would expect a NS/S ratio of 1.\n\n\n\nIn case of negative selection, NS/S is 1, and with positive selection, NS/S would be 1 (Biswas \u0026 Akey, 2006).\n\n\n\nFurthermore, the availability of large SNP datasets allowed us to determine where natural selection (either negative or positive) has effected variations in humans (Nielsen et al., 2007).\n\n\n\nIn this study, we investigated natural selection on the human genes by comparing the simple ratios of nonsynonymous and synonymous coding SNPs (cSNPs) in individual protein-coding genes.\n\n\n\nMethods.\n\n\n\nWe downloaded and analyzed all coding SNPs (cSNPs) with a validation code greater than 2 from the public dbSNP (build 125, http://www.ncbi.nlm.nih.gov/SNP/).\n\n\n\nWhere necessary, we additionally used genotype data generated from the International HapMap Project with udied the progression of chronic liver disease with regard to HCC.\n\n\n\nGenetic variations are thought to influence the risk of developing HCC (Edmondson, Henderson et al., 1976; Cha and Dematteo, 2005), particularly those that involve the activation of cellular oncogenes or the inactivation of tumor suppressor genes in various signaling pathways (e.g., mutation of beta-catenin-related Wnt/beta-catenin signals (Pang, Yuen et al., 2004) and overexpression of Ras signaling (Mitin, Rossman et al., 2005)).\n\n\n\nAlso, single nucleotide polymorphsims (SNPs) of many famous genes, such as p53 (Kirk, Lesi et al., 2005), HDAC10 (Park, Kim et al., 2007) and MMP2 (Wu, Zhang et al., 2008), have been significantly associated with HCC.\n\n\n\nThe significant SNPs of these genes may represent genetic markers that are in linkage disequilibrium (LD) with other causative variations.\n\n\n\nAlso recently, many SNP studies that are related to various human diseases have been reported (Lee, Kim et al., 2007).\n\n\n\nRas signaling transduction pathways influence cell proliferation, survival, differentiation, vesicular trafficking, and gene expression in B cells (Mitin, Rossman et al., 2005).\n\n\n\nIn previous studies, various growth factors were found to enhance HCC cell proliferation, as well as tu-C), and hepatitis B virus (HBV)-infected HCC.\n\n\n\nHCC is one of the most common malignant tumors worldwide and causes about 1 million deaths each year (Parkin et al., 2001; Marrero, 2006).\n\n\n\nThe etiology of HCC seems to be multifactorial, and several events appear to be necessary for malignant transformation to occur.\n\n\n\nHepatitis C virus (HCV) and HBV infections are important risk factors for chronic liver diseases (Collier \u0026 Sherman, 1998).\n\n\n\nCHB infection is the most common etiology of HCC in Asian countries.\n\n\n\nIn particular, cirrhosis is present in about 70% to 80% of HCC cases (Velazquez et al., 2003; Sy et al., 2005).\n\n\n\nMoreover, HCC is a highly hypervascular tumor that is associated with a high faculty for vascular invasion (Sun \u0026 Tang, 2004).\n\n\n\nBecause tumor angiogenesis plays a critical role in the development and progression of cancers, including HCC (Sun \u0026 Tang, 2004; Pang \u0026 Poon, 2007), angiogenic factors have been used not only for diagnosis and prognosis but also as predictors in cancer patients.\n\n\n\nn data or infer functional protein complexes from protein interaction data (Friedman et al., 2000).\n\n\n\nOthers combine various genomic data to infer biological networks without using prior knowledge about biological interaction.\n\n\n\nIn fact, there are a few recent works trying to reconstruct biological relations based on prior knowledge (Yamanishi et al., 2004; Kharchenko et al., 2004).\n\n\n\nYamanishi et al.\n\n\n\nuses kernel method to predict new gene-to-gene interaction within metabolic pathway and bases it on known pathway knowledge by adopting supervised approach.\n\n\n\nThe work of Kharchenko et al.\n\n\n\ncompares established metabolic network with expression profiles to find genes that can complete a metabolic pathway with some participants missed.\n\n\n\nWhile the methods are good in finding missing genes, they do not suggest possible new members (or genes) for the given biological pathway for pathway extension.\n\n\n\nWe first observe that a biological pathway contains highly verified information but covers only a small fraction of genes, while microarray data provide noisy experimental data but covers the whole genome.\n\n\n\nThe essence of PathPlus approach is to determine candidate genes that are highly likely to be related to a given pathway by combining microarray gene expression data n detail at any given moment.\n\n\n\nMoreover, most proteins are known to mediate their functions within regulated complex networks or pathways of interconnected macromolecules by forming dynamic topological interactomes.\n\n\n\nAdditionally, genes that are not significantly altered may play a critical role with other significantly dysregulated components in their biological pathways.\n\n\n\nTherefore, a systems biology approach that can identify pathways with these proteins would significantly improve the ability to find disease-associated genes from micorarray datasets.\n\n\n\nThis also would be useful in understanding the relationship between pathways and various phenotypes.\n\n\n\nThere has been a tremendous increase in information for constructing large-scale protein-protein interaction networks from public interactome databases, such as HPRD (Peri et al., 2004).\n\n\n\nA number of approaches have been demonstrated for identifying subnetworks of protein-protein interactions, based on coherent expression patterns of their genes (Chen and Yuan, 2006; Chuang et al., 2007).\n\n\n\nThere also is a study that has identified candidate genes that are related to certain diseases based only on the topological features of the network of disease-related protein-protein interactions (Hwang et al., 2008).\n\n\n\nRecently, several methods for integrating microarray data with metabolic pathways have been pre-sugar moieties, often deoxysugars, which add important features to the shape and the stereo-electronic properties of a molecule and often play an essential role in the biological activity of many natural product drugs (Rix et al., 2002).\n\n\n\nThus, glycosyltransferases are and will become more and more important tools for combinatorial biosynthetic approaches.\n\n\n\nIn this respect, our study focuses on developing the computational tools for the substrate prediction of a given glycosyltransferase (GT) and the prediction of deoxysuguar biosynthesis unit pathway.\n\n\n\nThe deoxysugar is synthesized by diverse biosynthetic enzymes and functions as a substrate of GTs.Features and Results.\n\n\n\nOur computational system developed in this work has 3-tier architecture which is composed of a client, an application server, and a back-end database server (Fig.\n\n\n\n1).\n\n\n\nThe application server consists of major three modules - pathway analysis module, GT analysis module and back-office module.\n\n\n\nThe pathway analysis module is involved in SBPD (Sugar Biosynthesis Pathway Database) search and sugar biosynthesis unit pathway prediction and drawing.\n\n\n\nThe main procedure of pathway ition of important functional groups to polyketide skeletons and to the structural diversity and biological activity of this class of natural products (Rix et al., 2002; Kwan et al., 2008).\n\n\n\nThe most frequently found post-PKS modifications are catalyzed by oxidoreductases, a very broad group of enzymes consisting of oxygenases, oxidases, peroxidases, reductases (e.g., ketoreductases), and dehydrogenases.\n\n\n\nIn general, these enzymes introduce oxygen-containing functionalities, such as hydroxyl groups (hydroxylases), aldehyde or keto groups, and epoxides (epoxidases) or modify such functionalities by addition or removal of hydrogen atoms, e.g.\n\n\n\ntransforming a ketone into a secondary alcohol or an aldehyde into a carboxylic acid.\n\n\n\nAlthough oxidoreductases provide or modify relatively small functional groups, they can have a tremendous impact on the binding properties of a molecule with respect to a biological ligand molecule (receptor protein, enzyme, DNA etc.).\n\n\n\nThe term group transferase refers to enzymes that possess transferase activity introducing novel functional groups and altered profiles on the product relative to the substrate.\n\n\n\nThis enzyme group contains important enzymes such as amino transferases, alkyl (usually methyl) transferases, acyl (usually acetyl) transferases, glycosyltransferases (GTs) rsity and background of complex diseases.\n\n\n\nFor defining CNV accurately, resolution is one of the important issues.\n\n\n\nWhen CNV was first uncovered, approximately 12 CNVs per genome were identified through both BAC array and oligoarray (Iafrate et al., 2004; Sebat et al., 2004).\n\n\n\nIn 2006, Affymetrix GeneChip Human Mapping 500K early access version was applied to define the CNVs from 269 HapMap individuals (Redon et al., 2006).\n\n\n\nIn that study, 1500 CNVs were identified and the median size of them was smaller (80 Kb) than those defined by tiling BAC array (230 Kb).\n\n\n\nIn addition to SNP-based CNV analysis, recent higher resolution oligoarray platforms were introduced and revealed that the human genome may contain more CNVs than previously thought and that the average size of CNVs might be smaller than previously reported (de Smith et al., 2007; Perry et al., 2008).\n\n\n\nIn spite of advance of new technologies, SNP marker has been used frequently to detect CNVs because of several advantages.\n\n\n\nFirst, due to large number of known SNP resources, extremely high resolution SNP genotyping chips (1 Million) can be designed and currently available.\n\n\n\nSecondly, accompanying SNP genotype information is useful for disease association study and CNV-SNP combined interpretation can achieve new breakthrough in understanding genetic contribution to oblems with incorporating recombination, the coalescent approach deals with individual sequences, which are different from the genotype data that we usually have.\n\n\n\nTherefore, in the coalescent approach, the stochastic inferences using genotype data involve additional assumptions or constraints (Zollner and Pritchard, 2005).\n\n\n\nThis problem continues when using sequenced data because the positions of mutation sites are given.\n\n\n\nIn order to find a possible future remedy for the current approaches and obtain more descriptive analyses from actual ancestral graphs instead of probability distributions of graphs, an alternative way of constructing ancestral graphs that avoids the multiple MRCA, as well as unnatural constraints, is proposed in this study.\n\n\n\nInstead of constructing the genealogies by coalescence of each individual sequence in a backward direction, the focus is on the emerging order of variants in the ancestral history of genetic data.\n\n\n\nBy concentrating on the variants themselves and constructing the graph in a forward direction, multiple MRCAs are avoidable, naturally lelic variation in the VDR gene explains 75% of the genetic variability in BMD used as a proxy measure (Eisman, 1999; Liu et al., 2003; Morrison et al., 1994).\n\n\n\nSince the first association by Morrison et al., allelic variations in genetic regulation of BMD have been subsequently studied in candidate genes related to important elements of bone mineral homeostasis, bone remodeling and bone matrix composition.\n\n\n\nThese approach were practically performed by restriction fragment length polymorphisms (RFLPs) on various populations: Caucasians (Deng et al., 1999; Langdahl et al., 2000; Quesada et al., 2004), African-Americans (Zmuda et al., 1999; Harris et al., 1997), Mexican-Americans (Kammerer et al., 2004; McClure et al., 1997), and Asians (Mitra et al., 2006; Morita et al., 2004; Yamada et al., 2003; Zhang et al., 2004).\n\n\n\nEthnicity was shown to be one of the important factors affecting BMD (Liel et al., 1988; Wang et al., 1997).\n\n\n\nHowever, controversial results of interethnic differences in allele or genotype distributions for BMD variation have been evidently presented, and a con-tir et al., 2000; Wolfe, 2000), which is an indicator of RA severity (Cabral et al., 2005).\n\n\n\nConsidering that the occurrence of RA reflects the interaction between genetic and environmental factors, an approach to estimating such interactions would be useful in the detection of RA susceptibility genes.\n\n\n\nA recent study reported a potential gene-environment interaction between the shared epitope of HLA-DR and smoking.\n\n\n\nIn RF-seropositive RA, HLA-DRB1 genotypes are associated with smoking, which means that a gene that is defined as a risk factor for RA is strongly affected by this environmental factor (Padyukov et al., 2004).\n\n\n\nWe hypothesized that smoking has a significant effect on the elevation of RA and that this relationship is mediated by the function of a specific gene.\n\n\n\nThis purpose of this study was to investigate the gene-environment interaction between smoking and the severity of RA.\n\n\n\nMethods .\n\n\n\nWe used the resources of the 15 Genetic Analysis Workshop (GAW 15) database and the North American Rheumatoid Arthritis Consortium (NARAC) family collec-arge heterooligomeric aggregates that protect other proteins against aggregation and denaturation (Mulder et al., 2007).\n\n\n\nHsp20 was first discovered in human skeletal muscle and has been found to be expressed in cardiac muscle, stomach, intestine and bladder (Rembold et al., 2000; Meeks et al., 2005; Mulder et al., 2007).\n\n\n\nThe phylum Proteobacteria is no exception in the sense that it too posses heat shock proteins.\n\n\n\nIt is one of the largest phyla of the Bacteria domain and is further subdivided into five categories: alpha, beta, delta, epsilon and gamma The alpha, beta and gamma subclasses were highly supported by morphological analyses, however, the delta and epsilon subclasses were added separately and are considered to have separated earlier than the other subclasses on the phylogenetic tree (Ludwig and Klenk, 2001).\n\n\n\nAll Proteobacteria are Gram negative and posses a membrane composed of lipopolysaccharides.\n\n\n\nBetaproteobacteria play a pivotal role in plant nitrogen fixation and are commonly found in environmental samples (Dedysh et al., 2004).\n\n\n\nEpsilonproteobacteria are known to inhabit the digestive tracts of animals and humans (Miroschnichenko et al., 2004).\n\n\n\nGammaproteobacteria encompass several species of medically and scientifically important groups of bacteria that include Salmonella, Vibrio, Pseudomonas and Escherichia (Lee et al., 2005).\n\n\n\nThe major aim of our present work is to study the sequence evolution of Hsp20 among all five subclasses of le way (Troyer, 2008).\n\n\n\nMany biobanks are currently in an establishment phase, aiming at collecting and archiving biomaterials and their relevant biomedical information for future use.\n\n\n\nOwing to this, several working protocols of sample collection, delivery, processing, storage, and retrieval are available for reference purposes (International Society for Biological Environmental Respositories 2008; Elliott and Peakman, 2008).\n\n\n\nHowever, the ultimate goal of a biobank is to make the general access of research-oriented people to high quality biomaterials possible with minimal efforts.\n\n\n\nThis requires an optimized procedure for sample distribution and proper measures of QA for archived biomaterials.\n\n\n\nFor instance, when genome-wide association studies which usually require tens of thousands of high quality DNA samples in a short period of time are underway, providing them with a proper level of QA in the required time frame can be a difficult task.\n\n\n\nQA of biomaterials in biobanks raises two issues: to authenticate that the specimen in a container matches exactly the participant that can be identified by the label on it and to avoid cross-contamination of biomaterials from different donors.\n\n\n\nAny of these QA breaches can mislead or nullify the research results in crucial ways.\n\n\n\nAlthough efforts to automate all or parts of biobanking projects in order to provide extensive genome data to the scientists.\n\n\n\nWe have also developed a user-friendly web site to facilitate access to sequences information of novel creatures.\n\n\n\nHere, we demonstrate a newly developed website, EKIS, which stands for EST Knowledge Integration System.\n\n\n\nThis website facilitates the annotation of new sequences with EC numbers and KEGG pathways based on BLASTX homology searches against the UniProt database using the PESTAS (http://pestas.\n\n\n\nkribb.re.kr/; unpublished).\n\n\n\nThe EKIS is utilized not only as a generic website for obtaining genome project statistics, such as samples, libraries, analyzing ESTs, and annotated ESTs in current ongoing projects, but also as a mining tool for the KEGG pathway based on annotated EC numbers, expression profiling, homology searches and retrieval information systems.\n\n\n\nFeatures and Results.\n\n\n\nThe EKIS, based on information derived from 65 EST sequencing projects (May 2008), is an integrated knowledge database developed using cutting-edge internet technology (Fig.\n\n\n\n1a).\n\n\n\nTo construct the data integrated ollection (WFCC), the World Data Center for Microorganism (WDCM), and the Asian Consortium for the Conservation and Sustainable Use of Microbial Resources (ACM).\n\n\n\nIn 2008, KACC developed a web-based system to provide an integrated database, which contains updated information on microbial resources.\n\n\n\nMethodology.\n\n\n\nThe database information was collected from the microorganism project (http://kacc.rda.go.kr/), the National Agrobiodiversity Center (NAC, http://genebank.rda.go.kr/), the National Academy of Agricultural Science (NAAS, http://www.niast.go.kr/), the Bio-Green 21 project (http:// biogreen21.rda.go.kr/), relevant microorganism divisions of universities, and various institutes in Korea.\n\n\n\nIn most instances, the strains were isolated from Korean agricultural environments, but some strains were shared with other culture collections.\n\n\n\nIn addition, the database information was accumulated and maintained through several collaborating international institutes, such as the Centraalbureau voor Schimmelcultures (CBS, http://www.\n\n\n\nhotgun fragment insert lengths.\n\n\n\nStill, these problems of the common assembly methods place a heavy burden on the bioinformaticians who work on the finishing stage of sequencing projects (Edwards et al., 1990).\n\n\n\nBesides these traditional difficulties, the recent technological divergence of high-throughput sequencing platforms, including 454 Life Sciences (Roche) (http:// www.454.com/), Solexa of the Illumina Genome Analysis System (http://www.illumina.com), and Applied Biosystems SOLiD Sequencing (http://ww.appliedbiosystems.\n\n\n\nUpon phosphorylation of Cbl, the Cbl/CAP complex provides docking sites for the recruitment of the adaptor protein CRKII and the guanyl nucleotide exchange factor C3G (Baumann et al., 2000; Ribon et al., 1996).\n\n\n\nRecruitment of C3G results in the activation of the small GTP-binding TC10 (protein product of the RHOQ gene).\n\n\n\nActivation of TC10 results in cytoskeletal rearrangements that are needed to facilitate Glut4 translocation upon insulin signaling as well as insulin-stimulated glucose uptake (Chiang et al., 2001).\n\n\n\nA recent study of streptozotocin-induced diabetic animals showed that Cbl and SORBS1 gene expression was significantly reduced and that the activation of TC10 was also abridged (Gupte and Mora, 2006).\n\n\n\nIn spite of the pivotal role of the CAP/TC10 pathway in skeletal muscle and adipose tissue, the association of effector genes with T2DM has not been elucidated well.\n\n\n\nIn the present report, we investigated the genetic var-kines such as TNFand interleukin-6 (IL-6) induce acute-phase proteins by the liver (Spranger et al., 2003).\n\n\n\nElevated levels of acute-phase proteins have been detected in T2DM subjects (McMillan, 1989; Jonsson and Wales, 1976).\n\n\n\nThese findings imply the relation of acute-phase proteins to T2DM through the action of proinflammatory agents.\n\n\n\nA variety of risk factors for T2DM development including age, inactivity, obesity, racial group, smoking, psychological stress, and low birth weight also have been known to be associated with augmented acute phase proteins (pickup, 2006).\n\n\n\nFibrinogen, one of the acute phase proteins, has been considered as a marker of cardiovascular disease (CVD) since it is the principal protein of blood clotting (Ernst \u0026 Resch, 1993).\n\n\n\nSome reports demonstrated linking plasma fibrinogen concentration to not only CVD but also the metabolic syndrome, namely T2DM, hypertriglyceridemia, hypertension, and hyperinsulinemia (Ganda \u0026 Arkin, 1992; Imperatore et al., 1998).\n\n\n\nFGA is A fast and cost-efficient approach to identify a multitude of novel SNPs involving in mining sequence data from public repositories needed to be developed.\n\n\n\nThe improvement of sequence trace data in public repositories at the DNA chromatogram level was required (Panitz et al., 2007).\n\n\n\nAn alternative and cheaper method of SNP identification exploits the redundancy of gene sequences generated by expressed sequence tags (ESTs; Gu et al., 1998).\n\n\n\n- In this scenario, each SNP would also be associated with an expressed gene.\n\n\n\nEST information has been used for the detection of SNPs in mammalian genomes by many groups (Buetow et al., 1999; Fitzsimmons et al., 2004; Garg et al., 1999; Guryev et al., 2004; Hawken et al., 2004; Kim et al., 2003; Lee et al., 2006a; Lee et al., 2006b; Picoult-Newberg, et al., 1999).\n\n\n\nMany conventional cDNA libraries have been constructed with porcine material.\n\n\n\nAs of May 2009, 1,532,429 ESTs were available in the GenBank porcine EST database and 167,740 SNPs were achievable in the GenBank pig SNP database (Table 1).\n\n\n\nSeveral researchers have reported mining cSNPs from porcine EST sequences (Dirisala et al., 2005, 2007; Fahrenkrug et al., 2002; Grapes et al., 2006; Kollers et al., 2005; Panitz et al., 2007; Park et al., 2009; Uenishi et al., e precursor cells exit the proliferation cycle and express young neuronal and glial characteristics.\n\n\n\nThe postmitotic cell bodies and differentiating cells are confined within the mantle layer and give rise to a distinct grey appearance.\n\n\n\nWithin this layer, some neurons develop local interconnections, while others elaborate axons to distal targets and form the marginal layer during the process.\n\n\n\nGlial cells gradually encapsulate the axons producing a marginal layer, which demonstrates a distinctive white appearance in the adult spinal cord.\n\n\n\nIn this way, neuronal and glial cells follow a developmental program that depends on temporal and regional patterning (Poh et al., 2002).\n\n\n\nRecent progress in the field has improved our understanding of the genetic control involved in neurogenesis and gliogenesis.\n\n\n\nFor example, the identification of the basic helix-loop-helix (bHLH) transcription factor has allowed us to further understand the molecular regulation of vertebrate neurogenesis and gliogenesis (Bertrand et al., 2002; Kageyama et al., 2005; Lu et al., 2000; Zhou and Anderson, 2002).\n\n\n\nDespite the identification of intrinsic cellular factors and extracellular cues, the molecular bases of general programs, including sequential generation, are not yet fully understood.\n\n\n\nIn this study, we employed microarray analysis to monitor the tempo-odels, EGCG causes a high mortality rate in adult female Swiss Webster mice and hepatotoxicity in immature C57BL/6 female mice (Galati et al., 2006; Goodin and Rosengren, 2003).\n\n\n\nAdditionally, recent studies have shown that several phenolic antioxidant food additives can accelerate oxidative damage to DNA, proteins, and carbohydrates, despite their antioxidative action.\n\n\n\nThe molecular mechanism of prooxidative action and hepatotoxicity that are induced by EGCG remains poorly understood.\n\n\n\nGalati et al.\n\n\n\n(2006) reported that cell death induced by EGCG was associated with increased production of reactive oxygen species (ROS) and depletion of reduced glutathione (GSH) and catechol-o-methyltransferase (COMT).\n\n\n\nThe mechanism of EGCG-induced cell death was suggested in previous reports, which implicated the apoptosis pathway (Schmidt et al.\n\n\n\n2005) and the endoplasmic reticulum (ER) stress-related pathway (Dodo et al., 2008).\n\n\n\nTo gain insight into the molecular mechanism underlying EGCG-induced cytotoxicity, we used a microarray approach, which allowed us to observe the global effects of EGCG on hepatic gene expression.\n\n\n\nTime course in this field of traditional Korean medicine is that both drug response and the disease susceptibility are related to a given persons particular psychological and physiological characteristics.\n\n\n\nBased on such characteristics, Sasang constitutional medicine classifies people into four constitutional types.\n\n\n\nIn the Sasang constitution type system, one is treated differently in medical procedures based on one’s type.\n\n\n\nIt is widely accepted in Korea that individualized medicine based on constitutional energy traits has a favorable influence on treatment (Leem and Park, 2007) and constitution-specific responses to herbs are well described (Jeong and Song, 1995a; Jeong and Song, 1995b).\n\n\n\nFurthermore inconsistent drug responses and disease susceptibility can be explained to some extent by characterizing the Sasang constitution and taking advantage of it in the course of treatment.\n\n\n\nStudies seeking genetic evidence for the Sasang constitution have been rare, except only a few reports showing an association between Sasang constitution type and specific genetic polymorphisms (Song et al., 2008; Um et al., 2003).\n\n\n\nTo unravel the genetic patterns of the Sasang constitution obtaining genetic evidence will be mandatory.\n\n\n\nAdvances in genetic analysis and statistical techni-d are essential for the organism's interaction with its environment.\n\n\n\nProteins such as channel proteins and transporters drive the transmembrane movement of ions, solutes, and small molecules.\n\n\n\nOther proteins such as receptors receive signals by interacting with a large variety of ligands, including hormones, neurotransmitters, autacoids, chemokines, odorants, even light, and transduce them to various kinds of information.\n\n\n\nMembrane proteins with seven transmembrane segments (7-TMSs) play major roles in signal transformation and information transport in cellular organisms.\n\n\n\nA wide variety of genomic and proteomic studies have been carried out since tens of whole genome sequences were determined.\n\n\n\nIn 2003, a database of experimentally characterized transmembrane topologies was constructed and reported as TMPDB; it contained a total of 302 TM protein sequences (Ikeda et al., 2003).\n\n\n\nRecently, the distributions of membrane protein topologies in both E. coli and S. cerevisiae membrane proteomes were reported (Daley et al., 2005; Kim et al., 2006).\n\n\n\nThere were a very small number of 7-TMS proteins compared with the numbers of proteins with other TMS numbers in E. coli, whereas a larger number of 7-TMS proteins were found in S. cerevisiae.\n\n\n\nInstead, E. approach that can identify perturbed molecular functions with differentially expressed genes would accelerate the understanding the basic molecular mechanism of certain phenotypes or diseases.\n\n\n\nThe Gene Ontology (The Gene Ontology Consortium, 2000) is a database of structured controlled ontology that describe gene product in terms of their biological processes, cellular components, and molecular functions.\n\n\n\nGO is a hierarchically structure forming an acyclic digraph with top-down directions, which provides an efficient navigation for the structure of the ontology.\n\n\n\nHowever, the same GO term may occur in different lines of ontology structure.\n\n\n\nIt means that GO may not be represented as directed tree since a GO term may have more than one parent providing multiple paths from the root.\n\n\n\nTo construct an ordered GO tree for the purpose of global visualization, GO terms need to be distinguished from one another if they are occurred in different locations on the hierarchical classification of gene ontology.\n\n\n\nBased on the biological point of view, Lee et al.\n\n\n\n(2004) justified that what is more important is not a GO term itself, but which path the GO terms takes from the root in the gene ontology.\n\n\n\nIt means that each location of a GO term could be considered distinct if a distinct path leads to it from the root of gene ontology.\n\n\n\nTherefore, we de-shuffling suggests that the two flanking introns of a domain-encoding exon should have symmetric phase combinations (Patthy, 1999).\n\n\n\nIntron phases are determined by the examination of the translational reading frame relative to the intron, and introns are thus described as phase 0, 1, or 2 introns.\n\n\n\nOf the nine possible combinations of flanking introns, three are symmetric (0-0, 1-1, and 2-2) and six are asymmetric.\n\n\n\nThe length of a symmetric exon is always a multiple of three nucleotides.\n\n\n\nOnly symmetric exons can be duplicated in tandem or deleted without affecting the reading frame, whereas the duplication and deletion of asymmetric exons can disrupt the downstream reading frame (Patthy, 1996).\n\n\n\nComparisons between human exon- boundary domains and bacterial domains have indicated that 1-1 domains are associated with the origin of animal multicellularity, whereas 0-0 domains are shared between eukaryotes and prokaryotes (Kaessmann, et al., 2002).\n\n\n\nThis comparison also indicates that 0-0 domains date back to before the prokaryote/eukaryote divergence and can thus be defined as ‘old domains’, whereas 1-1 domains were created recently and can thus be termed young domains.\n\n\n\nIn this study, we analyzed eight metazoan genomes rphism (SNP), because it implies that a smaller number of markers (tagging SNPs) are necessary to uniquely distinguish different haplotypes.\n\n\n\nOn the other hand, if a marker shows a strong association with a trait, then the other markers within the same haplotype block should show the same association.\n\n\n\nThis idea has been implemented in GWA analysis software programs, including PLINK (Purcell et al., 2007), in various ways: direct association of each haplotype, proxy association, or LD-based clumping.\n\n\n\nThese approaches require an accurate haplotype model.\n\n\n\nThe quality of the haplotype models in a particular GWA dataset can be improved by phasing the haplotypes, based on the background genotypes of the ultrahigh-density International HapMap data.\n\n\n\nThe International HapMap Project aims to produce such valuable haplotype information of the human genome (The International HapMap Consortium 2003).\n\n\n\nA total of 270 samples, 90 samples from each of three major ethnic groups-African, Asian, and European-were genotyped, phased, and released to the public freely (Thorisson et al., 2005).\n\n\n\nThe second phase of the project released the genotypes for over 2 million SNPs.\n\n\n\nThe genotypes of the markers that were not included in the study dataset but were included in the reference dataset, the International HapMap dataset, can be inferred if has been developed for eukaryotes by the group who developed COG (Koonin et al., 2004).\n\n\n\nInparanoid works by selecting orthologs by a reciprocal best hit, like COG.\n\n\n\nThe method also considers the existence of paralogs.\n\n\n\nTherefore, Inparanoid defines ortholog relations that are sometimes one-to-many or many-to-many (Remm et al., 2001).\n\n\n\nOrthoMCL (Li et al., 2003) is another kind of ortholog-finding system.\n\n\n\nThis method can find orthologs among several species at the same time, which was impossible for other methods.\n\n\n\nOrthoMCL uses an all- against-all BLAST search and a clustering algorithm based on the Markov model to select an ortholog group.\n\n\n\nHowever, these approaches above are basically using BLAST to check sequence similarity, and the proteins that have the best BLAST score are selected as the main orthologs.\n\n\n\nIt has been known that proteins that have the best similarity scores in BLAST search are often not the closest relatives phylogenetically (Koski and Golding, 2001).\n\n\n\nThis implies that there is a possibility that genuine orthologs can not be found using sequence similarity alone.\n\n\n\nNot only can sequence similarity be used for ortholog finding but also structure and interaction data.\n\n\n\nEven though the sequence similarity is not discovered, the including cases is high.\n\n\n\nIn such instances, we increase sample size for control only and then see if the effect on statistical power is the same as that obtained when the sample size for both case and control increases.\n\n\n\nSpecifically, we examine if increase in the ratio of control to case has an effect on increasing power.\n\n\n\nWe simulate SNP data as below and assess the effect of the ratio of control to case on statistical power.\n\n","tracks":[{"project":"Genomics_Informatics","denotations":[{"id":"LappsGridBioNER_protein1","span":{"begin":331,"end":335},"obj":"Protein"},{"id":"LappsGridBioNER_protein2","span":{"begin":346,"end":350},"obj":"Protein"},{"id":"LappsGridBioNER_protein3","span":{"begin":355,"end":370},"obj":"Protein"},{"id":"LappsGridBioNER_protein4","span":{"begin":690,"end":713},"obj":"Protein"},{"id":"LappsGridBioNER_protein5","span":{"begin":828,"end":846},"obj":"Protein"},{"id":"LappsGridBioNER_protein6","span":{"begin":988,"end":992},"obj":"Protein"},{"id":"LappsGridBioNER_protein7","span":{"begin":1186,"end":1215},"obj":"Protein"},{"id":"LappsGridBioNER_protein8","span":{"begin":2245,"end":2249},"obj":"Protein"},{"id":"LappsGridBioNER_protein9","span":{"begin":2471,"end":2478},"obj":"Protein"},{"id":"LappsGridBioNER_protein10","span":{"begin":4260,"end":4264},"obj":"Protein"},{"id":"LappsGridBioNER_protein11","span":{"begin":5285,"end":5289},"obj":"Protein"},{"id":"LappsGridBioNER_protein12","span":{"begin":5352,"end":5355},"obj":"Protein"},{"id":"LappsGridBioNER_protein13","span":{"begin":5865,"end":5876},"obj":"Protein"},{"id":"LappsGridBioNER_protein14","span":{"begin":6198,"end":6201},"obj":"Protein"},{"id":"LappsGridBioNER_protein15","span":{"begin":6693,"end":6697},"obj":"Protein"},{"id":"LappsGridBioNER_protein16","span":{"begin":6795,"end":6801},"obj":"Protein"},{"id":"LappsGridBioNER_protein17","span":{"begin":10780,"end":10784},"obj":"Protein"},{"id":"LappsGridBioNER_protein18","span":{"begin":10820,"end":10829},"obj":"Protein"},{"id":"LappsGridBioNER_protein19","span":{"begin":13761,"end":13765},"obj":"Protein"},{"id":"LappsGridBioNER_protein20","span":{"begin":14254,"end":14258},"obj":"Protein"},{"id":"LappsGridBioNER_protein21","span":{"begin":15026,"end":15030},"obj":"Protein"},{"id":"LappsGridBioNER_protein22","span":{"begin":16437,"end":16461},"obj":"Protein"},{"id":"LappsGridBioNER_protein23","span":{"begin":16470,"end":16495},"obj":"Protein"},{"id":"LappsGridBioNER_protein24","span":{"begin":16497,"end":16500},"obj":"Protein"},{"id":"LappsGridBioNER_protein25","span":{"begin":16506,"end":16530},"obj":"Protein"},{"id":"LappsGridBioNER_protein26","span":{"begin":17336,"end":17340},"obj":"Protein"},{"id":"LappsGridBioNER_protein27","span":{"begin":17446,"end":17457},"obj":"Protein"},{"id":"LappsGridBioNER_protein28","span":{"begin":19912,"end":19922},"obj":"Protein"},{"id":"LappsGridBioNER_protein29","span":{"begin":20678,"end":20696},"obj":"Protein"},{"id":"LappsGridBioNER_protein30","span":{"begin":27774,"end":27777},"obj":"Protein"},{"id":"LappsGridBioNER_protein31","span":{"begin":27992,"end":28014},"obj":"Protein"},{"id":"LappsGridBioNER_protein32","span":{"begin":28246,"end":28249},"obj":"Protein"},{"id":"LappsGridBioNER_protein33","span":{"begin":31140,"end":31143},"obj":"Protein"},{"id":"LappsGridBioNER_protein34","span":{"begin":33312,"end":33315},"obj":"Protein"},{"id":"LappsGridBioNER_protein35","span":{"begin":33356,"end":33359},"obj":"Protein"},{"id":"LappsGridBioNER_protein36","span":{"begin":33501,"end":33504},"obj":"Protein"},{"id":"LappsGridBioNER_protein37","span":{"begin":33635,"end":33638},"obj":"Protein"},{"id":"LappsGridBioNER_protein38","span":{"begin":33640,"end":33643},"obj":"Protein"},{"id":"LappsGridBioNER_protein39","span":{"begin":35969,"end":35997},"obj":"Protein"},{"id":"LappsGridBioNER_protein40","span":{"begin":39125,"end":39129},"obj":"Protein"},{"id":"LappsGridBioNER_protein41","span":{"begin":39287,"end":39305},"obj":"Protein"},{"id":"LappsGridBioNER_protein42","span":{"begin":39570,"end":39578},"obj":"Protein"},{"id":"LappsGridBioNER_protein43","span":{"begin":39834,"end":39852},"obj":"Protein"},{"id":"LappsGridBioNER_protein44","span":{"begin":40248,"end":40256},"obj":"Protein"},{"id":"LappsGridBioNER_protein45","span":{"begin":40357,"end":40367},"obj":"Protein"},{"id":"LappsGridBioNER_protein46","span":{"begin":40644,"end":40654},"obj":"Protein"},{"id":"LappsGridBioNER_protein47","span":{"begin":40835,"end":40838},"obj":"Protein"},{"id":"LappsGridBioNER_protein48","span":{"begin":41090,"end":41105},"obj":"Protein"},{"id":"LappsGridBioNER_protein49","span":{"begin":41309,"end":41318},"obj":"Protein"},{"id":"LappsGridBioNER_protein50","span":{"begin":41323,"end":41328},"obj":"Protein"},{"id":"LappsGridBioNER_protein51","span":{"begin":41399,"end":41422},"obj":"Protein"},{"id":"LappsGridBioNER_protein52","span":{"begin":41525,"end":41528},"obj":"Protein"},{"id":"LappsGridBioNER_protein53","span":{"begin":41667,"end":41690},"obj":"Protein"},{"id":"LappsGridBioNER_protein54","span":{"begin":41741,"end":41750},"obj":"Protein"},{"id":"LappsGridBioNER_protein55","span":{"begin":41755,"end":41760},"obj":"Protein"},{"id":"LappsGridBioNER_protein56","span":{"begin":41878,"end":41901},"obj":"Protein"},{"id":"LappsGridBioNER_protein57","span":{"begin":42193,"end":42207},"obj":"Protein"},{"id":"LappsGridBioNER_protein58","span":{"begin":42355,"end":42378},"obj":"Protein"},{"id":"LappsGridBioNER_protein59","span":{"begin":42455,"end":42474},"obj":"Protein"},{"id":"LappsGridBioNER_protein60","span":{"begin":43070,"end":43093},"obj":"Protein"},{"id":"LappsGridBioNER_protein61","span":{"begin":43174,"end":43177},"obj":"Protein"},{"id":"LappsGridBioNER_protein62","span":{"begin":43272,"end":43284},"obj":"Protein"},{"id":"LappsGridBioNER_protein63","span":{"begin":43576,"end":43596},"obj":"Protein"},{"id":"LappsGridBioNER_protein64","span":{"begin":43615,"end":43640},"obj":"Protein"},{"id":"LappsGridBioNER_protein65","span":{"begin":43701,"end":43712},"obj":"Protein"},{"id":"LappsGridBioNER_protein66","span":{"begin":43899,"end":43902},"obj":"Protein"},{"id":"LappsGridBioNER_protein67","span":{"begin":44105,"end":44110},"obj":"Protein"},{"id":"LappsGridBioNER_protein68","span":{"begin":44279,"end":44289},"obj":"Protein"},{"id":"LappsGridBioNER_protein69","span":{"begin":44378,"end":44382},"obj":"Protein"},{"id":"LappsGridBioNER_protein70","span":{"begin":44384,"end":44387},"obj":"Protein"},{"id":"LappsGridBioNER_protein71","span":{"begin":44389,"end":44394},"obj":"Protein"},{"id":"LappsGridBioNER_protein72","span":{"begin":44396,"end":44401},"obj":"Protein"},{"id":"LappsGridBioNER_protein73","span":{"begin":44407,"end":44413},"obj":"Protein"},{"id":"LappsGridBioNER_protein74","span":{"begin":46747,"end":46750},"obj":"Protein"},{"id":"LappsGridBioNER_protein75","span":{"begin":46791,"end":46794},"obj":"Protein"},{"id":"LappsGridBioNER_protein76","span":{"begin":46848,"end":46858},"obj":"Protein"},{"id":"LappsGridBioNER_protein77","span":{"begin":47041,"end":47044},"obj":"Protein"},{"id":"LappsGridBioNER_protein78","span":{"begin":47058,"end":47061},"obj":"Protein"},{"id":"LappsGridBioNER_protein79","span":{"begin":47194,"end":47197},"obj":"Protein"},{"id":"LappsGridBioNER_protein80","span":{"begin":47232,"end":47238},"obj":"Protein"},{"id":"LappsGridBioNER_protein81","span":{"begin":47545,"end":47548},"obj":"Protein"},{"id":"LappsGridBioNER_protein82","span":{"begin":48690,"end":48693},"obj":"Protein"},{"id":"LappsGridBioNER_protein83","span":{"begin":48906,"end":48912},"obj":"Protein"},{"id":"LappsGridBioNER_protein84","span":{"begin":49055,"end":49061},"obj":"Protein"},{"id":"LappsGridBioNER_protein85","span":{"begin":49179,"end":49185},"obj":"Protein"},{"id":"LappsGridBioNER_protein86","span":{"begin":49524,"end":49530},"obj":"Protein"},{"id":"LappsGridBioNER_protein87","span":{"begin":50492,"end":50498},"obj":"Protein"},{"id":"LappsGridBioNER_protein88","span":{"begin":50900,"end":50907},"obj":"Protein"},{"id":"LappsGridBioNER_protein89","span":{"begin":51992,"end":52000},"obj":"Protein"},{"id":"LappsGridBioNER_protein90","span":{"begin":53581,"end":53584},"obj":"Protein"},{"id":"LappsGridBioNER_protein91","span":{"begin":54263,"end":54277},"obj":"Protein"},{"id":"LappsGridBioNER_protein92","span":{"begin":54318,"end":54322},"obj":"Protein"},{"id":"LappsGridBioNER_protein93","span":{"begin":54345,"end":54363},"obj":"Protein"},{"id":"LappsGridBioNER_protein94","span":{"begin":54407,"end":54410},"obj":"Protein"},{"id":"LappsGridBioNER_protein95","span":{"begin":54566,"end":54580},"obj":"Protein"},{"id":"LappsGridBioNER_protein96","span":{"begin":54705,"end":54715},"obj":"Protein"},{"id":"LappsGridBioNER_protein97","span":{"begin":54821,"end":54835},"obj":"Protein"},{"id":"LappsGridBioNER_protein98","span":{"begin":54944,"end":54951},"obj":"Protein"},{"id":"LappsGridBioNER_protein99","span":{"begin":55013,"end":55016},"obj":"Protein"},{"id":"LappsGridBioNER_protein100","span":{"begin":55073,"end":55083},"obj":"Protein"},{"id":"LappsGridBioNER_protein101","span":{"begin":55100,"end":55104},"obj":"Protein"},{"id":"LappsGridBioNER_protein102","span":{"begin":55335,"end":55376},"obj":"Protein"},{"id":"LappsGridBioNER_protein103","span":{"begin":55378,"end":55382},"obj":"Protein"},{"id":"LappsGridBioNER_protein104","span":{"begin":55471,"end":55480},"obj":"Protein"},{"id":"LappsGridBioNER_protein105","span":{"begin":55639,"end":55643},"obj":"Protein"},{"id":"LappsGridBioNER_protein106","span":{"begin":56239,"end":56251},"obj":"Protein"},{"id":"LappsGridBioNER_protein107","span":{"begin":56272,"end":56275},"obj":"Protein"},{"id":"LappsGridBioNER_protein108","span":{"begin":56360,"end":56363},"obj":"Protein"},{"id":"LappsGridBioNER_protein109","span":{"begin":56472,"end":56482},"obj":"Protein"},{"id":"LappsGridBioNER_protein110","span":{"begin":56956,"end":56985},"obj":"Protein"},{"id":"LappsGridBioNER_protein111","span":{"begin":57188,"end":57217},"obj":"Protein"},{"id":"LappsGridBioNER_protein112","span":{"begin":57266,"end":57310},"obj":"Protein"},{"id":"LappsGridBioNER_protein113","span":{"begin":57408,"end":57430},"obj":"Protein"},{"id":"LappsGridBioNER_protein114","span":{"begin":57481,"end":57490},"obj":"Protein"},{"id":"LappsGridBioNER_protein115","span":{"begin":57636,"end":57644},"obj":"Protein"},{"id":"LappsGridBioNER_protein116","span":{"begin":57809,"end":57818},"obj":"Protein"},{"id":"LappsGridBioNER_protein117","span":{"begin":57876,"end":57885},"obj":"Protein"},{"id":"LappsGridBioNER_protein118","span":{"begin":58175,"end":58180},"obj":"Protein"},{"id":"LappsGridBioNER_protein119","span":{"begin":59654,"end":59657},"obj":"Protein"},{"id":"LappsGridBioNER_protein120","span":{"begin":60636,"end":60642},"obj":"Protein"},{"id":"LappsGridBioNER_protein121","span":{"begin":60660,"end":60665},"obj":"Protein"},{"id":"LappsGridBioNER_protein122","span":{"begin":60762,"end":60778},"obj":"Protein"},{"id":"LappsGridBioNER_protein123","span":{"begin":60965,"end":60975},"obj":"Protein"},{"id":"LappsGridBioNER_protein124","span":{"begin":61367,"end":61372},"obj":"Protein"},{"id":"LappsGridBioNER_protein125","span":{"begin":61452,"end":61456},"obj":"Protein"},{"id":"LappsGridBioNER_protein126","span":{"begin":61790,"end":61793},"obj":"Protein"},{"id":"LappsGridBioNER_protein127","span":{"begin":61838,"end":61843},"obj":"Protein"},{"id":"LappsGridBioNER_protein128","span":{"begin":62249,"end":62254},"obj":"Protein"},{"id":"LappsGridBioNER_protein129","span":{"begin":62347,"end":62352},"obj":"Protein"},{"id":"LappsGridBioNER_protein130","span":{"begin":62546,"end":62556},"obj":"Protein"},{"id":"LappsGridBioNER_protein131","span":{"begin":62596,"end":62599},"obj":"Protein"},{"id":"LappsGridBioNER_protein132","span":{"begin":62761,"end":62766},"obj":"Protein"},{"id":"LappsGridBioNER_protein133","span":{"begin":63151,"end":63187},"obj":"Protein"},{"id":"LappsGridBioNER_protein134","span":{"begin":64928,"end":64936},"obj":"Protein"},{"id":"LappsGridBioNER_protein135","span":{"begin":65058,"end":65066},"obj":"Protein"},{"id":"LappsGridBioNER_protein136","span":{"begin":65410,"end":65413},"obj":"Protein"},{"id":"LappsGridBioNER_protein137","span":{"begin":66108,"end":66112},"obj":"Protein"},{"id":"LappsGridBioNER_protein138","span":{"begin":66354,"end":66387},"obj":"Protein"},{"id":"LappsGridBioNER_protein139","span":{"begin":66389,"end":66393},"obj":"Protein"},{"id":"LappsGridBioNER_protein140","span":{"begin":66420,"end":66426},"obj":"Protein"},{"id":"LappsGridBioNER_protein141","span":{"begin":66428,"end":66433},"obj":"Protein"},{"id":"LappsGridBioNER_protein142","span":{"begin":66440,"end":66452},"obj":"Protein"},{"id":"LappsGridBioNER_protein143","span":{"begin":66454,"end":66458},"obj":"Protein"},{"id":"LappsGridBioNER_protein144","span":{"begin":67747,"end":67754},"obj":"Protein"},{"id":"LappsGridBioNER_protein145","span":{"begin":67794,"end":67819},"obj":"Protein"},{"id":"LappsGridBioNER_protein146","span":{"begin":70731,"end":70734},"obj":"Protein"},{"id":"LappsGridBioNER_protein147","span":{"begin":71412,"end":71415},"obj":"Protein"},{"id":"LappsGridBioNER_protein148","span":{"begin":74284,"end":74287},"obj":"Protein"},{"id":"LappsGridBioNER_protein149","span":{"begin":74652,"end":74670},"obj":"Protein"},{"id":"LappsGridBioNER_protein150","span":{"begin":74905,"end":74938},"obj":"Protein"},{"id":"LappsGridBioNER_protein151","span":{"begin":75205,"end":75226},"obj":"Protein"},{"id":"LappsGridBioNER_protein152","span":{"begin":75231,"end":75254},"obj":"Protein"},{"id":"LappsGridBioNER_protein153","span":{"begin":75256,"end":75259},"obj":"Protein"},{"id":"LappsGridBioNER_protein154","span":{"begin":75328,"end":75332},"obj":"Protein"},{"id":"LappsGridBioNER_protein155","span":{"begin":75554,"end":75563},"obj":"Protein"},{"id":"LappsGridBioNER_protein156","span":{"begin":76661,"end":76706},"obj":"Protein"},{"id":"LappsGridBioNER_protein157","span":{"begin":76755,"end":76758},"obj":"Protein"},{"id":"LappsGridBioNER_protein158","span":{"begin":76770,"end":76775},"obj":"Protein"},{"id":"LappsGridBioNER_protein159","span":{"begin":76879,"end":76882},"obj":"Protein"},{"id":"LappsGridBioNER_protein160","span":{"begin":76910,"end":76916},"obj":"Protein"},{"id":"LappsGridBioNER_protein161","span":{"begin":76946,"end":76950},"obj":"Protein"},{"id":"LappsGridBioNER_protein162","span":{"begin":77262,"end":77265},"obj":"Protein"},{"id":"LappsGridBioNER_protein163","span":{"begin":77289,"end":77292},"obj":"Protein"},{"id":"LappsGridBioNER_protein164","span":{"begin":77437,"end":77442},"obj":"Protein"},{"id":"LappsGridBioNER_protein165","span":{"begin":77499,"end":77513},"obj":"Protein"},{"id":"LappsGridBioNER_protein166","span":{"begin":77781,"end":77784},"obj":"Protein"},{"id":"LappsGridBioNER_protein167","span":{"begin":78221,"end":78224},"obj":"Protein"},{"id":"LappsGridBioNER_protein168","span":{"begin":78617,"end":78651},"obj":"Protein"},{"id":"LappsGridBioNER_protein169","span":{"begin":81508,"end":81511},"obj":"Protein"},{"id":"LappsGridBioNER_protein170","span":{"begin":81536,"end":81556},"obj":"Protein"},{"id":"LappsGridBioNER_protein171","span":{"begin":81766,"end":81785},"obj":"Protein"},{"id":"LappsGridBioNER_protein172","span":{"begin":82617,"end":82620},"obj":"Protein"},{"id":"LappsGridBioNER_protein173","span":{"begin":82785,"end":82795},"obj":"Protein"},{"id":"LappsGridBioNER_protein174","span":{"begin":82797,"end":82805},"obj":"Protein"},{"id":"LappsGridBioNER_protein175","span":{"begin":82807,"end":82830},"obj":"Protein"},{"id":"LappsGridBioNER_protein176","span":{"begin":82855,"end":82873},"obj":"Protein"},{"id":"LappsGridBioNER_protein177","span":{"begin":83399,"end":83425},"obj":"Protein"},{"id":"LappsGridBioNER_protein178","span":{"begin":83458,"end":83475},"obj":"Protein"},{"id":"LappsGridBioNER_protein179","span":{"begin":83499,"end":83518},"obj":"Protein"},{"id":"LappsGridBioNER_protein180","span":{"begin":83776,"end":83796},"obj":"Protein"},{"id":"LappsGridBioNER_protein181","span":{"begin":86283,"end":86291},"obj":"Protein"},{"id":"LappsGridBioNER_protein182","span":{"begin":87642,"end":87665},"obj":"Protein"},{"id":"LappsGridBioNER_protein183","span":{"begin":87765,"end":87771},"obj":"Protein"},{"id":"LappsGridBioNER_protein184","span":{"begin":87811,"end":87829},"obj":"Protein"},{"id":"LappsGridBioNER_protein185","span":{"begin":88390,"end":88436},"obj":"Protein"},{"id":"LappsGridBioNER_protein186","span":{"begin":88568,"end":88573},"obj":"Protein"},{"id":"LappsGridBioNER_protein187","span":{"begin":88847,"end":88866},"obj":"Protein"},{"id":"LappsGridBioNER_protein188","span":{"begin":88980,"end":89036},"obj":"Protein"},{"id":"LappsGridBioNER_protein189","span":{"begin":89120,"end":89127},"obj":"Protein"},{"id":"LappsGridBioNER_protein190","span":{"begin":89811,"end":89814},"obj":"Protein"},{"id":"LappsGridBioNER_protein191","span":{"begin":94024,"end":94027},"obj":"Protein"},{"id":"LappsGridBioNER_protein192","span":{"begin":94033,"end":94048},"obj":"Protein"},{"id":"LappsGridBioNER_protein193","span":{"begin":94099,"end":94120},"obj":"Protein"},{"id":"LappsGridBioNER_protein194","span":{"begin":94129,"end":94166},"obj":"Protein"},{"id":"LappsGridBioNER_protein195","span":{"begin":94229,"end":94232},"obj":"Protein"},{"id":"LappsGridBioNER_protein196","span":{"begin":94266,"end":94305},"obj":"Protein"},{"id":"LappsGridBioNER_protein197","span":{"begin":94313,"end":94322},"obj":"Protein"},{"id":"LappsGridBioNER_protein198","span":{"begin":94416,"end":94421},"obj":"Protein"},{"id":"LappsGridBioNER_protein199","span":{"begin":94441,"end":94448},"obj":"Protein"},{"id":"LappsGridBioNER_protein200","span":{"begin":94600,"end":94603},"obj":"Protein"},{"id":"LappsGridBioNER_protein201","span":{"begin":94608,"end":94619},"obj":"Protein"},{"id":"LappsGridBioNER_protein202","span":{"begin":94684,"end":94688},"obj":"Protein"},{"id":"LappsGridBioNER_protein203","span":{"begin":94770,"end":94786},"obj":"Protein"},{"id":"LappsGridBioNER_protein204","span":{"begin":94865,"end":94869},"obj":"Protein"},{"id":"LappsGridBioNER_protein205","span":{"begin":94972,"end":94992},"obj":"Protein"},{"id":"LappsGridBioNER_protein206","span":{"begin":94994,"end":94998},"obj":"Protein"},{"id":"LappsGridBioNER_protein207","span":{"begin":95252,"end":95256},"obj":"Protein"},{"id":"LappsGridBioNER_protein208","span":{"begin":95776,"end":95786},"obj":"Protein"},{"id":"LappsGridBioNER_protein209","span":{"begin":95971,"end":95974},"obj":"Protein"},{"id":"LappsGridBioNER_protein210","span":{"begin":96305,"end":96308},"obj":"Protein"},{"id":"LappsGridBioNER_protein211","span":{"begin":96563,"end":96567},"obj":"Protein"},{"id":"LappsGridBioNER_protein212","span":{"begin":97098,"end":97119},"obj":"Protein"},{"id":"LappsGridBioNER_protein213","span":{"begin":97949,"end":97952},"obj":"Protein"},{"id":"LappsGridBioNER_protein214","span":{"begin":98138,"end":98188},"obj":"Protein"},{"id":"LappsGridBioNER_protein215","span":{"begin":98650,"end":98654},"obj":"Protein"},{"id":"LappsGridBioNER_protein216","span":{"begin":99276,"end":99279},"obj":"Protein"},{"id":"LappsGridBioNER_protein217","span":{"begin":99328,"end":99356},"obj":"Protein"},{"id":"LappsGridBioNER_protein218","span":{"begin":99539,"end":99541},"obj":"Protein"},{"id":"LappsGridBioNER_protein219","span":{"begin":101956,"end":101976},"obj":"Protein"},{"id":"LappsGridBioNER_protein220","span":{"begin":102081,"end":102113},"obj":"Protein"},{"id":"LappsGridBioNER_protein221","span":{"begin":103447,"end":103450},"obj":"Protein"},{"id":"LappsGridBioNER_protein222","span":{"begin":104864,"end":104876},"obj":"Protein"},{"id":"LappsGridBioNER_protein223","span":{"begin":106908,"end":106916},"obj":"Protein"}],"attributes":[{"subj":"LappsGridBioNER_protein1","pred":"source","obj":"Genomics_Informatics"},{"subj":"LappsGridBioNER_protein2","pred":"source","obj":"Genomics_Informatics"},{"subj":"LappsGridBioNER_protein3","pred":"source","obj":"Genomics_Informatics"},{"subj":"LappsGridBioNER_protein4","pred":"source","obj":"Genomics_Informatics"},{"subj":"LappsGridBioNER_protein5","pred":"source","obj":"Genomics_Informatics"},{"subj":"LappsGridBioNER_protein6","pred":"source","obj":"Genomics_Informatics"},{"subj":"LappsGridBioNER_protein7","pred":"source","obj":"Genomics_Informatics"},{"subj":"LappsGridBioNER_protein8","pred":"source","obj":"Genomics_Informatics"},{"subj":"LappsGridBioNER_protein9","pred":"source","obj":"Genomics_Informatics"},{"subj":"LappsGridBioNER_protein10","pred":"source","obj":"Genomics_Informatics"},{"subj":"LappsGridBioNER_protein11","pred":"source","obj":"Genomics_Informatics"},{"subj":"LappsGridBioNER_protein12","pred":"source","obj":"Genomics_Informatics"},{"subj":"LappsGridBioNER_protein13","pred":"source","obj":"Genomics_Informatics"},{"subj":"LappsGridBioNER_protein14","pred":"source","obj":"Genomics_Informatics"},{"subj":"LappsGridBioNER_protein15","pred":"source","obj":"Genomics_Informatics"},{"subj":"LappsGridBioNER_protein16","pred":"source","obj":"Genomics_Informatics"},{"subj":"LappsGridBioNER_protein17","pred":"source","obj":"Genomics_Informatics"},{"subj":"LappsGridBioNER_protein18","pred":"source","obj":"Genomics_Informatics"},{"subj":"LappsGridBioNER_protein19","pred":"source","obj":"Genomics_Informatics"},{"subj":"LappsGridBioNER_protein20","pred":"source","obj":"Genomics_Informatics"},{"subj":"LappsGridBioNER_protein21","pred":"source","obj":"Genomics_Informatics"},{"subj":"LappsGridBioNER_protein22","pred":"source","obj":"Genomics_Informatics"},{"subj":"LappsGridBioNER_protein23","pred":"source","obj":"Genomics_Informatics"},{"subj":"LappsGridBioNER_protein24","pred":"source","obj":"Genomics_Informatics"},{"subj":"LappsGridBioNER_protein25","pred":"source","obj":"Genomics_Informatics"},{"subj":"LappsGridBioNER_protein26","pred":"source","obj":"Genomics_Informatics"},{"subj":"LappsGridBioNER_protein27","pred":"source","obj":"Genomics_Informatics"},{"subj":"LappsGridBioNER_protein28","pred":"source","obj":"Genomics_Informatics"},{"subj":"LappsGridBioNER_protein29","pred":"source","obj":"Genomics_Informatics"},{"subj":"LappsGridBioNER_protein30","pred":"source","obj":"Genomics_Informatics"},{"subj":"LappsGridBioNER_protein31","pred":"source","obj":"Genomics_Informatics"},{"subj":"LappsGridBioNER_protein32","pred":"source","obj":"Genomics_Informatics"},{"subj":"LappsGridBioNER_protein33","pred":"source","obj":"Genomics_Informatics"},{"subj":"LappsGridBioNER_protein34","pred":"source","obj":"Genomics_Informatics"},{"subj":"LappsGridBioNER_protein35","pred":"source","obj":"Genomics_Informatics"},{"subj":"LappsGridBioNER_protein36","pred":"source","obj":"Genomics_Informatics"},{"subj":"LappsGridBioNER_protein37","pred":"source","obj":"Genomics_Informatics"},{"subj":"LappsGridBioNER_protein38","pred":"source","obj":"Genomics_Informatics"},{"subj":"LappsGridBioNER_protein39","pred":"source","obj":"Genomics_Informatics"},{"subj":"LappsGridBioNER_protein40","pred":"source","obj":"Genomics_Informatics"},{"subj":"LappsGridBioNER_protein41","pred":"source","obj":"Genomics_Informatics"},{"subj":"LappsGridBioNER_protein42","pred":"source","obj":"Genomics_Informatics"},{"subj":"LappsGridBioNER_protein43","pred":"source","obj":"Genomics_Informatics"},{"subj":"LappsGridBioNER_protein44","pred":"source","obj":"Genomics_Informatics"},{"subj":"LappsGridBioNER_protein45","pred":"source","obj":"Genomics_Informatics"},{"subj":"LappsGridBioNER_protein46","pred":"source","obj":"Genomics_Informatics"},{"subj":"LappsGridBioNER_protein47","pred":"source","obj":"Genomics_Informatics"},{"subj":"LappsGridBioNER_protein48","pred":"source","obj":"Genomics_Informatics"},{"subj":"LappsGridBioNER_protein49","pred":"source","obj":"Genomics_Informatics"},{"subj":"LappsGridBioNER_protein50","pred":"source","obj":"Genomics_Informatics"},{"subj":"LappsGridBioNER_protein51","pred":"source","obj":"Genomics_Informatics"},{"subj":"LappsGridBioNER_protein52","pred":"source","obj":"Genomics_Informatics"},{"subj":"LappsGridBioNER_protein53","pred":"source","obj":"Genomics_Informatics"},{"subj":"LappsGridBioNER_protein54","pred":"source","obj":"Genomics_Informatics"},{"subj":"LappsGridBioNER_protein55","pred":"source","obj":"Genomics_Informatics"},{"subj":"LappsGridBioNER_protein56","pred":"source","obj":"Genomics_Informatics"},{"subj":"LappsGridBioNER_protein57","pred":"source","obj":"Genomics_Informatics"},{"subj":"LappsGridBioNER_protein58","pred":"source","obj":"Genomics_Informatics"},{"subj":"LappsGridBioNER_protein59","pred":"source","obj":"Genomics_Informatics"},{"subj":"LappsGridBioNER_protein60","pred":"source","obj":"Genomics_Informatics"},{"subj":"LappsGridBioNER_protein61","pred":"source","obj":"Genomics_Informatics"},{"subj":"LappsGridBioNER_protein62","pred":"source","obj":"Genomics_Informatics"},{"subj":"LappsGridBioNER_protein63","pred":"source","obj":"Genomics_Informatics"},{"subj":"LappsGridBioNER_protein64","pred":"source","obj":"Genomics_Informatics"},{"subj":"LappsGridBioNER_protein65","pred":"source","obj":"Genomics_Informatics"},{"subj":"LappsGridBioNER_protein66","pred":"source","obj":"Genomics_Informatics"},{"subj":"LappsGridBioNER_protein67","pred":"source","obj":"Genomics_Informatics"},{"subj":"LappsGridBioNER_protein68","pred":"source","obj":"Genomics_Informatics"},{"subj":"LappsGridBioNER_protein69","pred":"source","obj":"Genomics_Informatics"},{"subj":"LappsGridBioNER_protein70","pred":"source","obj":"Genomics_Informatics"},{"subj":"LappsGridBioNER_protein71","pred":"source","obj":"Genomics_Informatics"},{"subj":"LappsGridBioNER_protein72","pred":"source","obj":"Genomics_Informatics"},{"subj":"LappsGridBioNER_protein73","pred":"source","obj":"Genomics_Informatics"},{"subj":"LappsGridBioNER_protein74","pred":"source","obj":"Genomics_Informatics"},{"subj":"LappsGridBioNER_protein75","pred":"source","obj":"Genomics_Informatics"},{"subj":"LappsGridBioNER_protein76","pred":"source","obj":"Genomics_Informatics"},{"subj":"LappsGridBioNER_protein77","pred":"source","obj":"Genomics_Informatics"},{"subj":"LappsGridBioNER_protein78","pred":"source","obj":"Genomics_Informatics"},{"subj":"LappsGridBioNER_protein79","pred":"source","obj":"Genomics_Informatics"},{"subj":"LappsGridBioNER_protein80","pred":"source","obj":"Genomics_Informatics"},{"subj":"LappsGridBioNER_protein81","pred":"source","obj":"Genomics_Informatics"},{"subj":"LappsGridBioNER_protein82","pred":"source","obj":"Genomics_Informatics"},{"subj":"LappsGridBioNER_protein83","pred":"source","obj":"Genomics_Informatics"},{"subj":"LappsGridBioNER_protein84","pred":"source","obj":"Genomics_Informatics"},{"subj":"LappsGridBioNER_protein85","pred":"source","obj":"Genomics_Informatics"},{"subj":"LappsGridBioNER_protein86","pred":"source","obj":"Genomics_Informatics"},{"subj":"LappsGridBioNER_protein87","pred":"source","obj":"Genomics_Informatics"},{"subj":"LappsGridBioNER_protein88","pred":"source","obj":"Genomics_Informatics"},{"subj":"LappsGridBioNER_protein89","pred":"source","obj":"Genomics_Informatics"},{"subj":"LappsGridBioNER_protein90","pred":"source","obj":"Genomics_Informatics"},{"subj":"LappsGridBioNER_protein91","pred":"source","obj":"Genomics_Informatics"},{"subj":"LappsGridBioNER_protein92","pred":"source","obj":"Genomics_Informatics"},{"subj":"LappsGridBioNER_protein93","pred":"source","obj":"Genomics_Informatics"},{"subj":"LappsGridBioNER_protein94","pred":"source","obj":"Genomics_Informatics"},{"subj":"LappsGridBioNER_protein95","pred":"source","obj":"Genomics_Informatics"},{"subj":"LappsGridBioNER_protein96","pred":"source","obj":"Genomics_Informatics"},{"subj":"LappsGridBioNER_protein97","pred":"source","obj":"Genomics_Informatics"},{"subj":"LappsGridBioNER_protein98","pred":"source","obj":"Genomics_Informatics"},{"subj":"LappsGridBioNER_protein99","pred":"source","obj":"Genomics_Informatics"},{"subj":"LappsGridBioNER_protein100","pred":"source","obj":"Genomics_Informatics"},{"subj":"LappsGridBioNER_protein101","pred":"source","obj":"Genomics_Informatics"},{"subj":"LappsGridBioNER_protein102","pred":"source","obj":"Genomics_Informatics"},{"subj":"LappsGridBioNER_protein103","pred":"source","obj":"Genomics_Informatics"},{"subj":"LappsGridBioNER_protein104","pred":"source","obj":"Genomics_Informatics"},{"subj":"LappsGridBioNER_protein105","pred":"source","obj":"Genomics_Informatics"},{"subj":"LappsGridBioNER_protein106","pred":"source","obj":"Genomics_Informatics"},{"subj":"LappsGridBioNER_protein107","pred":"source","obj":"Genomics_Informatics"},{"subj":"LappsGridBioNER_protein108","pred":"source","obj":"Genomics_Informatics"},{"subj":"LappsGridBioNER_protein109","pred":"source","obj":"Genomics_Informatics"},{"subj":"LappsGridBioNER_protein110","pred":"source","obj":"Genomics_Informatics"},{"subj":"LappsGridBioNER_protein111","pred":"source","obj":"Genomics_Informatics"},{"subj":"LappsGridBioNER_protein112","pred":"source","obj":"Genomics_Informatics"},{"subj":"LappsGridBioNER_protein113","pred":"source","obj":"Genomics_Informatics"},{"subj":"LappsGridBioNER_protein114","pred":"source","obj":"Genomics_Informatics"},{"subj":"LappsGridBioNER_protein115","pred":"source","obj":"Genomics_Informatics"},{"subj":"LappsGridBioNER_protein116","pred":"source","obj":"Genomics_Informatics"},{"subj":"LappsGridBioNER_protein117","pred":"source","obj":"Genomics_Informatics"},{"subj":"LappsGridBioNER_protein118","pred":"source","obj":"Genomics_Informatics"},{"subj":"LappsGridBioNER_protein119","pred":"source","obj":"Genomics_Informatics"},{"subj":"LappsGridBioNER_protein120","pred":"source","obj":"Genomics_Informatics"},{"subj":"LappsGridBioNER_protein121","pred":"source","obj":"Genomics_Informatics"},{"subj":"LappsGridBioNER_protein122","pred":"source","obj":"Genomics_Informatics"},{"subj":"LappsGridBioNER_protein123","pred":"source","obj":"Genomics_Informatics"},{"subj":"LappsGridBioNER_protein124","pred":"source","obj":"Genomics_Informatics"},{"subj":"LappsGridBioNER_protein125","pred":"source","obj":"Genomics_Informatics"},{"subj":"LappsGridBioNER_protein126","pred":"source","obj":"Genomics_Informatics"},{"subj":"LappsGridBioNER_protein127","pred":"source","obj":"Genomics_Informatics"},{"subj":"LappsGridBioNER_protein128","pred":"source","obj":"Genomics_Informatics"},{"subj":"LappsGridBioNER_protein129","pred":"source","obj":"Genomics_Informatics"},{"subj":"LappsGridBioNER_protein130","pred":"source","obj":"Genomics_Informatics"},{"subj":"LappsGridBioNER_protein131","pred":"source","obj":"Genomics_Informatics"},{"subj":"LappsGridBioNER_protein132","pred":"source","obj":"Genomics_Informatics"},{"subj":"LappsGridBioNER_protein133","pred":"source","obj":"Genomics_Informatics"},{"subj":"LappsGridBioNER_protein134","pred":"source","obj":"Genomics_Informatics"},{"subj":"LappsGridBioNER_protein135","pred":"source","obj":"Genomics_Informatics"},{"subj":"LappsGridBioNER_protein136","pred":"source","obj":"Genomics_Informatics"},{"subj":"LappsGridBioNER_protein137","pred":"source","obj":"Genomics_Informatics"},{"subj":"LappsGridBioNER_protein138","pred":"source","obj":"Genomics_Informatics"},{"subj":"LappsGridBioNER_protein139","pred":"source","obj":"Genomics_Informatics"},{"subj":"LappsGridBioNER_protein140","pred":"source","obj":"Genomics_Informatics"},{"subj":"LappsGridBioNER_protein141","pred":"source","obj":"Genomics_Informatics"},{"subj":"LappsGridBioNER_protein142","pred":"source","obj":"Genomics_Informatics"},{"subj":"LappsGridBioNER_protein143","pred":"source","obj":"Genomics_Informatics"},{"subj":"LappsGridBioNER_protein144","pred":"source","obj":"Genomics_Informatics"},{"subj":"LappsGridBioNER_protein145","pred":"source","obj":"Genomics_Informatics"},{"subj":"LappsGridBioNER_protein146","pred":"source","obj":"Genomics_Informatics"},{"subj":"LappsGridBioNER_protein147","pred":"source","obj":"Genomics_Informatics"},{"subj":"LappsGridBioNER_protein148","pred":"source","obj":"Genomics_Informatics"},{"subj":"LappsGridBioNER_protein149","pred":"source","obj":"Genomics_Informatics"},{"subj":"LappsGridBioNER_protein150","pred":"source","obj":"Genomics_Informatics"},{"subj":"LappsGridBioNER_protein151","pred":"source","obj":"Genomics_Informatics"},{"subj":"LappsGridBioNER_protein152","pred":"source","obj":"Genomics_Informatics"},{"subj":"LappsGridBioNER_protein153","pred":"source","obj":"Genomics_Informatics"},{"subj":"LappsGridBioNER_protein154","pred":"source","obj":"Genomics_Informatics"},{"subj":"LappsGridBioNER_protein155","pred":"source","obj":"Genomics_Informatics"},{"subj":"LappsGridBioNER_protein156","pred":"source","obj":"Genomics_Informatics"},{"subj":"LappsGridBioNER_protein157","pred":"source","obj":"Genomics_Informatics"},{"subj":"LappsGridBioNER_protein158","pred":"source","obj":"Genomics_Informatics"},{"subj":"LappsGridBioNER_protein159","pred":"source","obj":"Genomics_Informatics"},{"subj":"LappsGridBioNER_protein160","pred":"source","obj":"Genomics_Informatics"},{"subj":"LappsGridBioNER_protein161","pred":"source","obj":"Genomics_Informatics"},{"subj":"LappsGridBioNER_protein162","pred":"source","obj":"Genomics_Informatics"},{"subj":"LappsGridBioNER_protein163","pred":"source","obj":"Genomics_Informatics"},{"subj":"LappsGridBioNER_protein164","pred":"source","obj":"Genomics_Informatics"},{"subj":"LappsGridBioNER_protein165","pred":"source","obj":"Genomics_Informatics"},{"subj":"LappsGridBioNER_protein166","pred":"source","obj":"Genomics_Informatics"},{"subj":"LappsGridBioNER_protein167","pred":"source","obj":"Genomics_Informatics"},{"subj":"LappsGridBioNER_protein168","pred":"source","obj":"Genomics_Informatics"},{"subj":"LappsGridBioNER_protein169","pred":"source","obj":"Genomics_Informatics"},{"subj":"LappsGridBioNER_protein170","pred":"source","obj":"Genomics_Informatics"},{"subj":"LappsGridBioNER_protein171","pred":"source","obj":"Genomics_Informatics"},{"subj":"LappsGridBioNER_protein172","pred":"source","obj":"Genomics_Informatics"},{"subj":"LappsGridBioNER_protein173","pred":"source","obj":"Genomics_Informatics"},{"subj":"LappsGridBioNER_protein174","pred":"source","obj":"Genomics_Informatics"},{"subj":"LappsGridBioNER_protein175","pred":"source","obj":"Genomics_Informatics"},{"subj":"LappsGridBioNER_protein176","pred":"source","obj":"Genomics_Informatics"},{"subj":"LappsGridBioNER_protein177","pred":"source","obj":"Genomics_Informatics"},{"subj":"LappsGridBioNER_protein178","pred":"source","obj":"Genomics_Informatics"},{"subj":"LappsGridBioNER_protein179","pred":"source","obj":"Genomics_Informatics"},{"subj":"LappsGridBioNER_protein180","pred":"source","obj":"Genomics_Informatics"},{"subj":"LappsGridBioNER_protein181","pred":"source","obj":"Genomics_Informatics"},{"subj":"LappsGridBioNER_protein182","pred":"source","obj":"Genomics_Informatics"},{"subj":"LappsGridBioNER_protein183","pred":"source","obj":"Genomics_Informatics"},{"subj":"LappsGridBioNER_protein184","pred":"source","obj":"Genomics_Informatics"},{"subj":"LappsGridBioNER_protein185","pred":"source","obj":"Genomics_Informatics"},{"subj":"LappsGridBioNER_protein186","pred":"source","obj":"Genomics_Informatics"},{"subj":"LappsGridBioNER_protein187","pred":"source","obj":"Genomics_Informatics"},{"subj":"LappsGridBioNER_protein188","pred":"source","obj":"Genomics_Informatics"},{"subj":"LappsGridBioNER_protein189","pred":"source","obj":"Genomics_Informatics"},{"subj":"LappsGridBioNER_protein190","pred":"source","obj":"Genomics_Informatics"},{"subj":"LappsGridBioNER_protein191","pred":"source","obj":"Genomics_Informatics"},{"subj":"LappsGridBioNER_protein192","pred":"source","obj":"Genomics_Informatics"},{"subj":"LappsGridBioNER_protein193","pred":"source","obj":"Genomics_Informatics"},{"subj":"LappsGridBioNER_protein194","pred":"source","obj":"Genomics_Informatics"},{"subj":"LappsGridBioNER_protein195","pred":"source","obj":"Genomics_Informatics"},{"subj":"LappsGridBioNER_protein196","pred":"source","obj":"Genomics_Informatics"},{"subj":"LappsGridBioNER_protein197","pred":"source","obj":"Genomics_Informatics"},{"subj":"LappsGridBioNER_protein198","pred":"source","obj":"Genomics_Informatics"},{"subj":"LappsGridBioNER_protein199","pred":"source","obj":"Genomics_Informatics"},{"subj":"LappsGridBioNER_protein200","pred":"source","obj":"Genomics_Informatics"},{"subj":"LappsGridBioNER_protein201","pred":"source","obj":"Genomics_Informatics"},{"subj":"LappsGridBioNER_protein202","pred":"source","obj":"Genomics_Informatics"},{"subj":"LappsGridBioNER_protein203","pred":"source","obj":"Genomics_Informatics"},{"subj":"LappsGridBioNER_protein204","pred":"source","obj":"Genomics_Informatics"},{"subj":"LappsGridBioNER_protein205","pred":"source","obj":"Genomics_Informatics"},{"subj":"LappsGridBioNER_protein206","pred":"source","obj":"Genomics_Informatics"},{"subj":"LappsGridBioNER_protein207","pred":"source","obj":"Genomics_Informatics"},{"subj":"LappsGridBioNER_protein208","pred":"source","obj":"Genomics_Informatics"},{"subj":"LappsGridBioNER_protein209","pred":"source","obj":"Genomics_Informatics"},{"subj":"LappsGridBioNER_protein210","pred":"source","obj":"Genomics_Informatics"},{"subj":"LappsGridBioNER_protein211","pred":"source","obj":"Genomics_Informatics"},{"subj":"LappsGridBioNER_protein212","pred":"source","obj":"Genomics_Informatics"},{"subj":"LappsGridBioNER_protein213","pred":"source","obj":"Genomics_Informatics"},{"subj":"LappsGridBioNER_protein214","pred":"source","obj":"Genomics_Informatics"},{"subj":"LappsGridBioNER_protein215","pred":"source","obj":"Genomics_Informatics"},{"subj":"LappsGridBioNER_protein216","pred":"source","obj":"Genomics_Informatics"},{"subj":"LappsGridBioNER_protein217","pred":"source","obj":"Genomics_Informatics"},{"subj":"LappsGridBioNER_protein218","pred":"source","obj":"Genomics_Informatics"},{"subj":"LappsGridBioNER_protein219","pred":"source","obj":"Genomics_Informatics"},{"subj":"LappsGridBioNER_protein220","pred":"source","obj":"Genomics_Informatics"},{"subj":"LappsGridBioNER_protein221","pred":"source","obj":"Genomics_Informatics"},{"subj":"LappsGridBioNER_protein222","pred":"source","obj":"Genomics_Informatics"},{"subj":"LappsGridBioNER_protein223","pred":"source","obj":"Genomics_Informatics"}]}],"config":{"attribute types":[{"pred":"source","value type":"selection","values":[{"id":"Genomics_Informatics","color":"#93ece8","default":true}]}]}}